如果你也在 怎样代写贝叶斯分析Bayesian Analysis这个学科遇到相关的难题,请随时右上角联系我们的24/7代写客服。贝叶斯分析Bayesian Analysis自1763年以来,我们现在所知道的贝叶斯统计学并没有一个明确的运行。尽管贝叶斯的方法被拉普拉斯和当时其他领先的概率论者热情地接受,但在19世纪却陷入了不光彩的境地,因为他们还不知道如何正确处理先验概率。20世纪上半叶,一种完全不同的理论得到了发展,现在称为频繁主义统计学。但贝叶斯思想的火焰被少数思想家保持着,如意大利的布鲁诺-德-菲内蒂和英国的哈罗德-杰弗里斯。
贝叶斯分析Bayesian Analysis现代贝叶斯运动开始于20世纪下半叶,由美国的Jimmy Savage和英国的Dennis Lindley带头,但贝叶斯推断仍然极难实现,直到20世纪80年代末和90年代初,强大的计算机开始广泛使用,新的计算方法被开发出来。随后,人们对贝叶斯统计的兴趣大增,不仅导致了贝叶斯方法论的广泛研究,也导致了使用贝叶斯方法来解决天体物理学、天气预报、医疗保健政策和刑事司法等不同应用领域的迫切问题。
my-assignmentexpert™贝叶斯分析Bayesian Analysis代写,免费提交作业要求, 满意后付款,成绩80\%以下全额退款,安全省心无顾虑。专业硕 博写手团队,所有订单可靠准时,保证 100% 原创。my-assignmentexpert™, 最高质量的贝叶斯分析Bayesian Analysis作业代写,服务覆盖北美、欧洲、澳洲等 国家。 在代写价格方面,考虑到同学们的经济条件,在保障代写质量的前提下,我们为客户提供最合理的价格。 由于统计Statistics作业种类很多,同时其中的大部分作业在字数上都没有具体要求,因此贝叶斯分析Bayesian Analysis作业代写的价格不固定。通常在经济学专家查看完作业要求之后会给出报价。作业难度和截止日期对价格也有很大的影响。
想知道您作业确定的价格吗? 免费下单以相关学科的专家能了解具体的要求之后在1-3个小时就提出价格。专家的 报价比上列的价格能便宜好几倍。
my-assignmentexpert™ 为您的留学生涯保驾护航 在澳洲代写方面已经树立了自己的口碑, 保证靠谱, 高质且原创的澳洲代写服务。我们的专家在贝叶斯分析Bayesian Analysis代写方面经验极为丰富,各种贝叶斯分析Bayesian Analysis相关的作业也就用不着 说。
我们提供的贝叶斯分析Bayesian Analysis及其相关学科的代写,服务范围广, 其中包括但不限于:
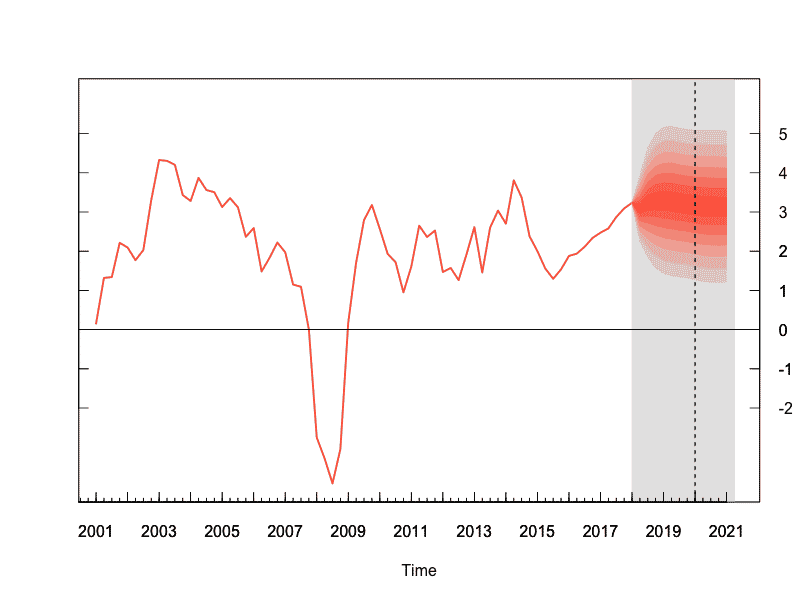
澳洲代考|贝叶斯分析代考Bayesian Analysis 代考|Introduction
This book presents the Bayesian approach to the analysis of time series and illustrates the techniques related to this with many examples. Using the software packages $R$ and WinBUGS enhances the way a Bayesian approach can be implemented. The $R$ package is primarily used to generate observations from a given time series model, while the WinBUGS package allows one to perform a posterior analysis that provides the way to determine the characteristic of the posterior distribution of the unknown parameters. It is important to realize that the reader most likely would not execute the Bayesian analysis provided by the author. This is because the investigator would have different prior information and possibly a different likelihood for a given sample information. Many of the examples are based on data generated by $\mathrm{R}$ and the author places noninformative prior distribution on the parameters. It is also important to note that the posterior analysis executed by WinBUGS would also most likely be different than the analyses that appear in the book. This is due to the fact that others would possibly use a different number of observations for the Monte Carlo Markov Chain (MCMC) simulation with different prior distributions and different initial values for the parameters. There are not many books on time series from a Bayesian point of view, but the author has based his approach to some extent on earlier textbooks such as Pole and West, ${ }^{1}$ West and Harrison, ${ }^{2}$ Spall, ${ }^{3}$ and Venkatesan and Aramugam. ${ }^{4}$ I have also relied heavily on the books by Broemeling ${ }^{5,6}$ that present some of the earliest ideas on Bayesian time series.
My approach in this book is presented in roughly three phases: (1) First is the data that are sometimes generated by $R$ from a time series whose parameters are known or data from a scientific investigation, (2) a specification of the model and prior distribution of the unknown parameters, and (3) the posterior analysis either presented analytically or numerically by WinBUGS. My book is somewhat unique in that it uses $\mathrm{R}$ and WinBUGS to expedite the analysis of a given series.
澳洲代考|贝叶斯分析代考Bayesian Analysis 代考|Chapter 2: Bayesian Inference and MCMC Simulation
The chapter begins with a description of Bayes theorem followed by the four phases of statistical inference, namely: (1) prior information, (2) sample information represented by the likelihood function, (3) the joint posterior distribution of the parameters, and (4) if appropriate, forecasting future observations via the Bayesian predictive density ( or mass function). Bayesian inferential techniques are illustrated with many examples. For example, the first population to be considered is the binomial with one parameter, the probability of success in $n$ independent trials, where a beta prior is placed on the parameter, which when combined with the likelihood function gives a beta posterior distribution for the probability of success. Based on the posterior distribution of the parameter, point estimation, and interval estimation are described, and finally the Bayesian predictive mass function is derived for $\mathrm{m}$ future independent trials, and demonstrations for the binomial population is done with $n=11$ trials and $m=5$ future trials. The same four-phase scenario is explained for the normal, multinomial, and Poisson populations.
The remaining sections of the chapter are devoted to the description of MCMC algorithms for the simulation of observations from the relevant posterior distributions. Also included in the concluding sections of the chapter are detailed directions for how to use $\mathrm{R}$ and WinBUGS.
澳洲代考|贝叶斯分析代考BAYESIAN ANALYSIS 代考|Chapter 3: Fundamentals of Time Series Analysis
A time series is defined as a stochastic process whose index is time, and several examples are explained:
(1) Airline passenger bookings over a 10-year period, (2) the sunspot cycle, and (3). Annual Los Angeles rainfall data, starting in 1903 . The first two series have an increasing trend over time and clearly have seasonal components, while the third (the rainfall data) has a constant trend and no obvious seasonal effects. At this point, the student is shown how to employ $\mathrm{R}$ to graph the data and how to delineate the series into trend and seasonal components. The $R$ package has a function called decomposition that portrays the observations, the trend of the series, the seasonal component, and the errors of a given series. Decomposition is a powerful tool and should always be used when analyzing a time series. This will give the investigator enough information to propose a tentative time series model. Also presented are definitions of the mean value function and variance function of the time series, the autocorrelation function, and the correlogram. These characteristic of a time series are computed for the airline passenger booking data, the sunspot cycle data, and the Los Angeles rainfall data using the appropriate $\mathrm{R}$ command (e.g. mean(), $\operatorname{std}()$, autocorrelation or $\operatorname{acf}()$, and partial autocorrelation or pacf()).
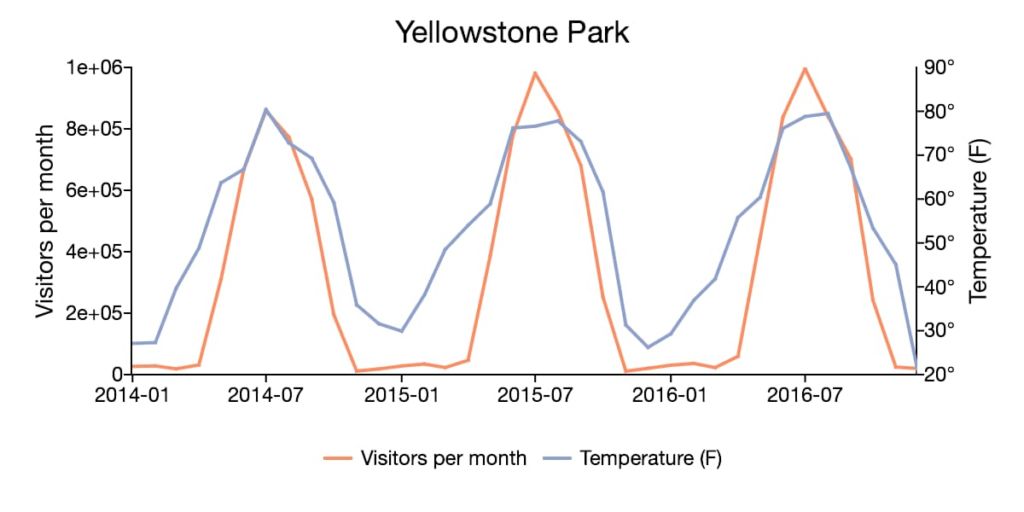
贝叶斯分析代写
澳洲代考|贝叶斯分析代考BAYESIAN ANALYSIS 代考|INTRODUCTION
本书介绍了时间序列分析的贝叶斯方法,并通过许多示例说明了与此相关的技术。使用软件包RWinBUGS 增强了贝叶斯方法的实现方式。这R软件包主要用于从给定的时间序列模型生成观察结果,而 WinBUGS 软件包允许执行后验分析,提供确定未知参数后验分布特征的方法。重要的是要意识到读者很可能不会执行作者提供的贝叶斯分析。这是因为调查人员将有不同的先验信息,并且可能对给定的样本信息有不同的可能性。许多示例都基于由R并且作者在参数上放置了非信息性的先验分布。同样重要的是要注意 WinBUGS 执行的后验分析也很可能与书中出现的分析不同。这是因为其他人可能会对蒙特卡洛马尔可夫链使用不同数量的观察值米C米C具有不同的先验分布和不同的参数初始值的模拟。从贝叶斯的角度来看,关于时间序列的书籍并不多,但作者在某种程度上基于 Pole 和 West 等早期教科书的方法,1韦斯特和哈里森,2剥落,3还有文卡特桑和阿拉穆甘。4我也非常依赖 Broemeling 的书5,6提出了关于贝叶斯时间序列的一些最早的想法。
我在本书中的方法大致分为三个阶段:1首先是有时生成的数据R来自参数已知的时间序列或来自科学研究的数据,2模型的规范和未知参数的先验分布,以及3WinBUGS 以分析或数值方式呈现后验分析。我的书有些独特之处在于它使用R和 WinBUGS 以加快对给定系列的分析。
澳洲代考|贝叶斯分析代考BAYESIAN ANALYSIS 代考|CHAPTER 2: BAYESIAN INFERENCE AND MCMC SIMULATION
本章首先描述贝叶斯定理,然后是统计推断的四个阶段,即:1先验信息,2似然函数表示的样本信息,3参数的联合后验分布,以及4如果合适,通过贝叶斯预测密度预测未来的观察结果○r米一个ssF在nC吨一世○n. 贝叶斯推理技术用许多例子来说明。例如,要考虑的第一个总体是具有一个参数的二项式,即在n独立试验,其中将 beta 先验放在参数上,当与似然函数结合时,会给出成功概率的 beta 后验分布。基于参数的后验分布,描述点估计和区间估计,最后推导出贝叶斯预测质量函数米未来的独立试验,以及对二项式总体的演示n=11试验和米=5未来的试验。对正态、多项式和泊松总体解释了相同的四阶段情景。
本章的其余部分专门描述 MCMC 算法,用于模拟来自相关后验分布的观察结果。本章的结尾部分还包括如何使用的详细说明R和 WinBUGS。
澳洲代考|贝叶斯分析代考BAYESIAN ANALYSIS 代考|CHAPTER 3: FUNDAMENTALS OF TIME SERIES ANALYSIS
时间序列被定义为一个以时间为指标的随机过程,并解释了几个例子:
1过去 10 年的航空公司乘客预订量,2太阳黑子周期,和3. 洛杉矶年降雨量数据,始于 1903 年。前两个系列随着时间的推移呈上升趋势,并且明显具有季节性成分,而第三个系列吨H和r一个一世nF一个lld一个吨一个具有恒定的趋势,没有明显的季节性影响。在这一点上,向学生展示了如何使用R将数据绘制成图表,以及如何将系列描绘成趋势和季节性成分。这R包有一个称为分解的函数,它描述了观测值、序列的趋势、季节性分量和给定序列的误差。分解是一种强大的工具,在分析时间序列时应始终使用。这将为调查人员提供足够的信息来提出暂定的时间序列模型。还介绍了时间序列的均值函数和方差函数、自相关函数和相关图的定义。使用适当的方法为航空公司乘客预订数据、太阳黑子周期数据和洛杉矶降雨数据计算时间序列的这些特征R命令和.G.米和一个n(, 性病(), 自相关或acf(), 和偏自相关或 pacf).
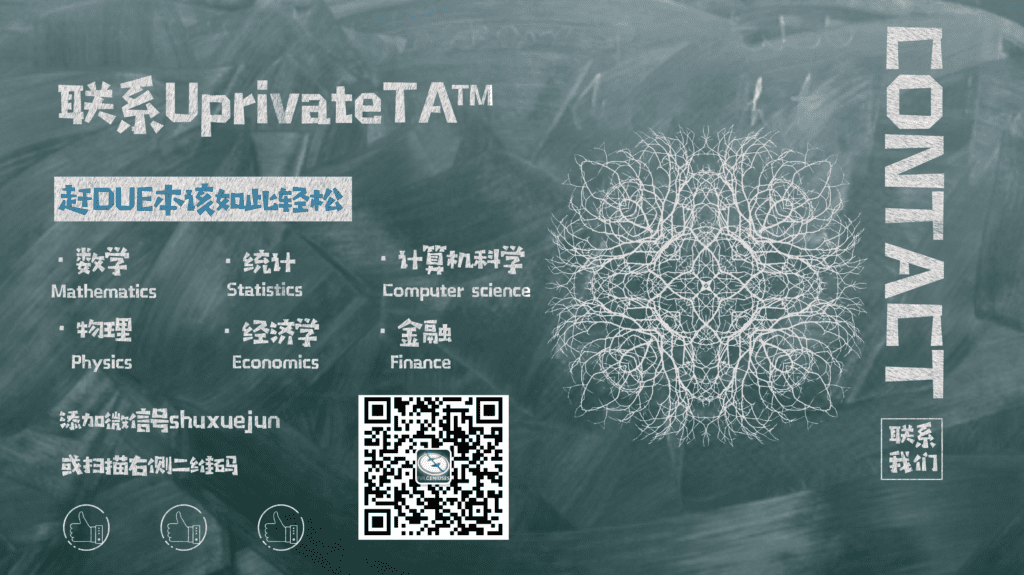
澳洲代考|贝叶斯分析代考Bayesian Analysis 代考 请认准UprivateTA™. UprivateTA™为您的留学生涯保驾护航。
微观经济学代写
微观经济学是主流经济学的一个分支,研究个人和企业在做出有关稀缺资源分配的决策时的行为以及这些个人和企业之间的相互作用。my-assignmentexpert™ 为您的留学生涯保驾护航 在数学Mathematics作业代写方面已经树立了自己的口碑, 保证靠谱, 高质且原创的数学Mathematics代写服务。我们的专家在图论代写Graph Theory代写方面经验极为丰富,各种图论代写Graph Theory相关的作业也就用不着 说。
线性代数代写
线性代数是数学的一个分支,涉及线性方程,如:线性图,如:以及它们在向量空间和通过矩阵的表示。线性代数是几乎所有数学领域的核心。
博弈论代写
现代博弈论始于约翰-冯-诺伊曼(John von Neumann)提出的两人零和博弈中的混合策略均衡的观点及其证明。冯-诺依曼的原始证明使用了关于连续映射到紧凑凸集的布劳威尔定点定理,这成为博弈论和数学经济学的标准方法。在他的论文之后,1944年,他与奥斯卡-莫根斯特恩(Oskar Morgenstern)共同撰写了《游戏和经济行为理论》一书,该书考虑了几个参与者的合作游戏。这本书的第二版提供了预期效用的公理理论,使数理统计学家和经济学家能够处理不确定性下的决策。
微积分代写
微积分,最初被称为无穷小微积分或 “无穷小的微积分”,是对连续变化的数学研究,就像几何学是对形状的研究,而代数是对算术运算的概括研究一样。
它有两个主要分支,微分和积分;微分涉及瞬时变化率和曲线的斜率,而积分涉及数量的累积,以及曲线下或曲线之间的面积。这两个分支通过微积分的基本定理相互联系,它们利用了无限序列和无限级数收敛到一个明确定义的极限的基本概念 。
计量经济学代写
什么是计量经济学?
计量经济学是统计学和数学模型的定量应用,使用数据来发展理论或测试经济学中的现有假设,并根据历史数据预测未来趋势。它对现实世界的数据进行统计试验,然后将结果与被测试的理论进行比较和对比。
根据你是对测试现有理论感兴趣,还是对利用现有数据在这些观察的基础上提出新的假设感兴趣,计量经济学可以细分为两大类:理论和应用。那些经常从事这种实践的人通常被称为计量经济学家。
Matlab代写
MATLAB 是一种用于技术计算的高性能语言。它将计算、可视化和编程集成在一个易于使用的环境中,其中问题和解决方案以熟悉的数学符号表示。典型用途包括:数学和计算算法开发建模、仿真和原型制作数据分析、探索和可视化科学和工程图形应用程序开发,包括图形用户界面构建MATLAB 是一个交互式系统,其基本数据元素是一个不需要维度的数组。这使您可以解决许多技术计算问题,尤其是那些具有矩阵和向量公式的问题,而只需用 C 或 Fortran 等标量非交互式语言编写程序所需的时间的一小部分。MATLAB 名称代表矩阵实验室。MATLAB 最初的编写目的是提供对由 LINPACK 和 EISPACK 项目开发的矩阵软件的轻松访问,这两个项目共同代表了矩阵计算软件的最新技术。MATLAB 经过多年的发展,得到了许多用户的投入。在大学环境中,它是数学、工程和科学入门和高级课程的标准教学工具。在工业领域,MATLAB 是高效研究、开发和分析的首选工具。MATLAB 具有一系列称为工具箱的特定于应用程序的解决方案。对于大多数 MATLAB 用户来说非常重要,工具箱允许您学习和应用专业技术。工具箱是 MATLAB 函数(M 文件)的综合集合,可扩展 MATLAB 环境以解决特定类别的问题。可用工具箱的领域包括信号处理、控制系统、神经网络、模糊逻辑、小波、仿真等。