如果你也在 怎样代写贝叶斯分析Bayesian Analysis STA421这个学科遇到相关的难题,请随时右上角联系我们的24/7代写客服。贝叶斯分析Bayesian Analysis简单地说,在任何你有大量异质或噪音数据的应用领域,或者你需要清楚了解你的不确定性的地方,都是你可以使用贝叶斯统计的领域。
贝叶斯分析Bayesian Analysis一种统计推断方法(以英国数学家托马斯-贝叶斯命名),它允许人们将关于人口参数的先验信息与样本中包含的信息证据相结合,以指导统计推断过程。首先指定一个感兴趣的参数的先验概率分布。然后通过应用贝叶斯定理获得并结合证据,为参数提供一个后验概率分布。后验分布为有关该参数的统计推断提供了基础。
my-assignmentexpert™贝叶斯分析Bayesian Analysis代写,免费提交作业要求, 满意后付款,成绩80\%以下全额退款,安全省心无顾虑。专业硕 博写手团队,所有订单可靠准时,保证 100% 原创。my-assignmentexpert™, 最高质量的贝叶斯分析Bayesian Analysis作业代写,服务覆盖北美、欧洲、澳洲等 国家。 在代写价格方面,考虑到同学们的经济条件,在保障代写质量的前提下,我们为客户提供最合理的价格。 由于统计Statistics作业种类很多,同时其中的大部分作业在字数上都没有具体要求,因此贝叶斯分析Bayesian Analysis作业代写的价格不固定。通常在经济学专家查看完作业要求之后会给出报价。作业难度和截止日期对价格也有很大的影响。
想知道您作业确定的价格吗? 免费下单以相关学科的专家能了解具体的要求之后在1-3个小时就提出价格。专家的 报价比上列的价格能便宜好几倍。
my-assignmentexpert™ 为您的留学生涯保驾护航 在统计代写方面已经树立了自己的口碑, 保证靠谱, 高质且原创的统计代考服务。我们的专家在贝叶斯分析Bayesian Analysis代写方面经验极为丰富,各种贝叶斯分析Bayesian Analysis相关的作业也就用不着 说。
我们提供的贝叶斯分析Bayesian Analysis STA421及其相关学科的代写,服务范围广, 其中包括但不限于:
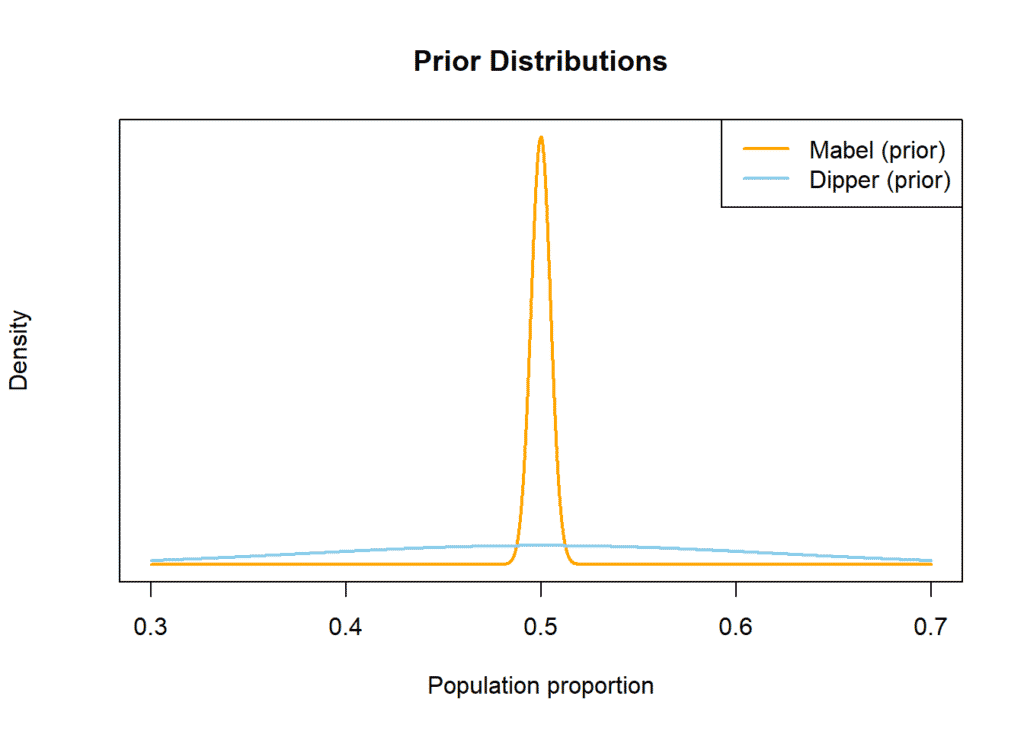
统计代写|贝叶斯分析代考Bayesian Analysis 代考|Introduction
It is imperative to check model adequacy in order to choose an appropriate model and to conduct a valid study. The approach taken here is based on many sources, including Gelman et al., ${ }^{14}$ Carlin and Louis, 18 and Congdon ${ }^{16}$ Our main focus will be on the likelihood function of the posterior distribution, and not the prior distribution, and to this end, graphical representations such as histograms, boxplots, and various probability plots of the original observations will be compared with those of the observations generated from the predictive distribution. In addition to graphical methods, Bayesian versions of overall goodness-offit-type operations are taken to check model validity. Methods presented at this juncture are just a small subset of those presented in more advanced works, including Gelman et al., Carlin and Louis, and Congdon.
Of course, the prior distribution is an important component of the analysis, and if one is not sure of the ‘true’ prior, one should perform a sensitivity analysis to determine the robustness of posterior inferences to various alternative choices of prior information. See Gelman et al. or Carlin and Louis for details of performing a sensitivity study for prior information. Our approach is to either use informative or vague prior distributions, where the former is done when prior relevant experimental evidence determines the prior, or the latter is taken if there is none or very little germane experimental studies. In scientific studies, the most likely scenario is that there are relevant experimental studies providing informative prior information.
统计代写|贝叶斯分析代考Bayesian Analysis 代考|Sampling from an Exponential, but Assuming a Normal Population
Consider a random sample of size 30 from an exponential distribution with mean 3 . An exponential distribution is often used to model the survival times of a screening test. The 30 exponential values are generated with BC $2.4$.
BC $2.4$
model;
${$ for $(\mathrm{i}$ in $1: 30){$
$X[i] \sim \operatorname{dexp}(3)$
}$}$
$\mathrm{x}=(1 \cdot 9075,0.7683,5 \cdot 8364,3 \cdot 0821,0.0276,15 \cdot 0444,2.3591,14 \cdot 9290,6 \cdot 3841,7 \cdot 6572,$,
$5.9606,1 \cdot 5316,3 \cdot 1619,1 \cdot 5236,2 \cdot 5458,1 \cdot 6693,4 \cdot 2076,6 \cdot 7704,7 \cdot 0414,1 \cdot 0895,3.7661$,
$0.0673,1 \cdot 3952,2.8778,5.8272,1 \cdot 5335,7.2606,3 \cdot 1171,4$
The sample mean and standard deviation are $4.13$ and $3.739$, respectively.
Assume the sample is from a normal population with unknown mean and variance, with an improper prior density
$\xi(\mu, \tau)=1 / \tau, \mu \in R$ and $\sigma>0$, then the posterior predictive density is a univariate with $n-1=29$ degrees of freedom, mean $\bar{x}=3.744$, standard deviation $3.872$, and precision $p=.0645$. This is verified from the original observations $x$ and the formula for the precision. From the predictive distribution, 30 observations are generated with BC $2.5$.
BC 2.5.
{
For $(t$ in $1: 30){$
$\mathrm{Z}[\mathrm{t}] \mathrm{t}(3.744, .0645,29)}$
}
$\mathrm{z}=(2 \cdot 76213,3 \cdot 46370,2 \cdot 88747,3 \cdot 13581,4 \cdot 50398,5 \cdot 09963,4 \cdot 39670,3 \cdot 24032,3 \cdot 58791,$,
$5.60893,3 \cdot 76411,3 \cdot 15034,4 \cdot 15961,2 \cdot 83306,3 \cdot 64620,3 \cdot 48478,2 \cdot 24699,2 \cdot 44810$,
$3.39590,3.56703,4 \cdot 04226,4 \cdot 00720,4 \cdot 33006,3 \cdot 44320,5 \cdot 03451,2 \cdot 07679,2.30578$,
$5.99297,3.88463,2.52737)$
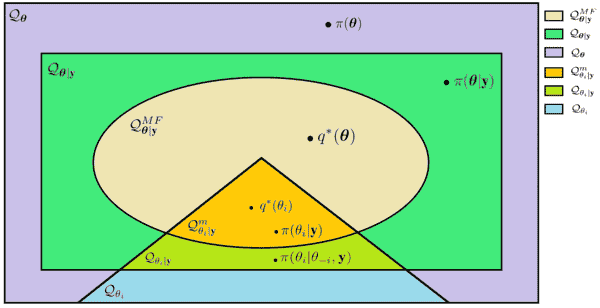
贝叶斯分析代写
统计代写|贝叶斯分析代考BAYESIAN ANALYSIS 代 考|INTRODUCTION
为了选择合适的模型并进行有效的研究,必须检查模型的充分性。这里采用的方法基于许多来源,包括 Gelman等人, 14 卡林和路易斯,18岁和康登 16 我们的主要关注 点将放在后验分布的似然函数上,而不是先验分布上,为此,原始观测值的直方图、箱线图和各种概率图等图形表示将与生成的观测值进行比较从预则分布。除了图形 方法之外,还采用贝叶斯版本的整体优劣型操作来检查模型的有效性。此时提出的方法只是更高级作品中提出的方法的一小部分,包括 Gelman 等人、Carlin 和 Louis以 及 Congdon。
当然,先验分布是分析的重要组成部分,如果不确定“真实”先验,则应进行敏感性分析,以确定后验推断对各种先验信息选择的稳健侏。见 Gelman 等人。或 Carlin 和 Louis,了解对先验信息进行敏感性研究的详细信息。我们的方法是使用信息丰富或模楜的先验分布,其中前者是在先前的相关实验证据确定先验时完成的,或者在没 有或很少有密切相关的实验研究时采用后者。在科学研究中,最可能的情兄是相关的实验研究提供了丰富的先验信息。
统计代写|贝叶斯分析代考BAYESIAN ANALYSIS 代考|SAMPLING FROM AN EXPONENTIAL, BUT ASSUMING A NORMAL POPULATION
考虑来自均值为 3 的指数分布的大小为 30 的随机样本。指数分布通常用于模拟筛选测试的生存时间。 30 个指数值是用 $B C$ 生成的 $2.4$ 。
公元前 $2.4$
模型;
$\$$ for $\$(1 \$ i n \$ 1: 30) \$ \$ X[i] \sim \operatorname{dexp}(3) \$ \$$
$\mathrm{x}=(1 \cdot 9075,0.7683,5 \cdot 8364,3 \cdot 0821,0.0276,15 \cdot 0444,2.3591,14 \cdot 9290,6 \cdot 3841,7 \cdot 6572,$,
$5.9606,1 \cdot 5316,3 \cdot 1619,1 \cdot 5236,2 \cdot 5458,1 \cdot 6693,4 \cdot 2076,6 \cdot 7704,7 \cdot 0414,1 \cdot 0895,3.7661$
$0.0673,1 \cdot 3952,2.8778,5.8272,1 \cdot 5335,7.2606,3 \cdot 1171,4$
样本均值和标准差为 $4.13$ 和 $3.739$ ,分别。
假设样本来自具有末知均值和方差的正态总体,具有不正确的先验密度
$\xi(\mu, \tau)=1 / \tau, \mu \in R$ 和 $\sigma>0$, 那么后验预测密度是一个单变量 $n-1=29$ 自由度,平均值 $x=3.744$, 标准差 $3.872$, 和精度 $p=.0645$. 这从原始观察中得到验证 $x$ 以
及精度公式。根据预测分布,使用 $B C$ 生成 30 个观测值 $2.5$.
公元前 2.5。
i
对于 $(t$ 在 $1: 30) \$ \$ Z[t] \mathrm{t}(3.744, .0645,29)$
$\mathrm{z}=(2 \cdot 76213,3 \cdot 46370,2 \cdot 88747,3 \cdot 13581,4 \cdot 50398,5 \cdot 09963,4 \cdot 39670,3 \cdot 24032,3 \cdot 58791,$,
$5.60893,3 \cdot 76411,3 \cdot 15034,4 \cdot 15961,2 \cdot 83306,3 \cdot 64620,3 \cdot 48478,2 \cdot 24699,2 \cdot 44810$
$3.39590,3.56703,4 \cdot 04226,4 \cdot 00720,4 \cdot 33006,3 \cdot 44320,5 \cdot 03451,2 \cdot 07679,2.30578$
$5.99297,3.88463,2.52737)$
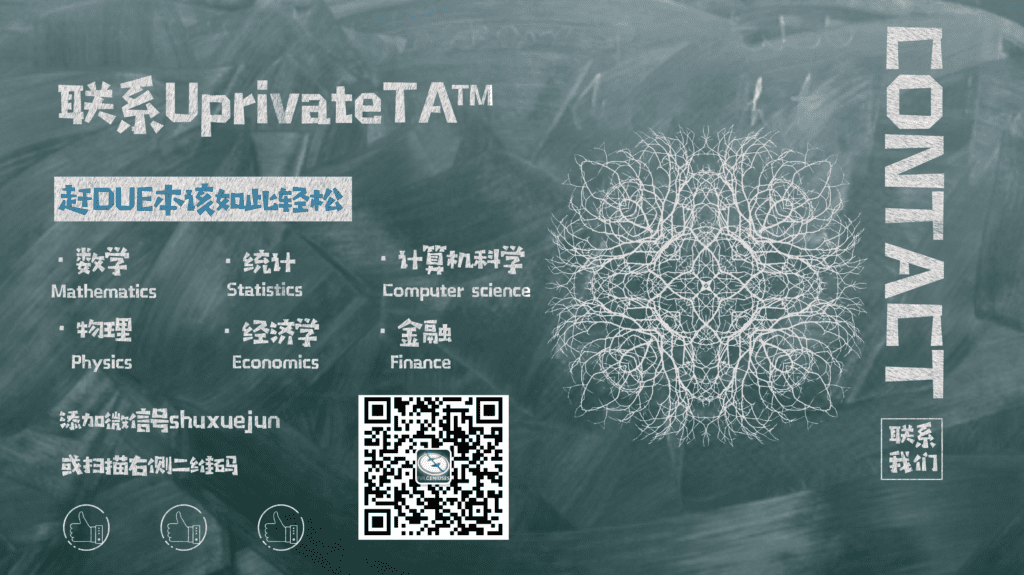
统计代写|贝叶斯分析代考Bayesian Analysis 代考 请认准UprivateTA™. UprivateTA™为您的留学生涯保驾护航。
微观经济学代写
微观经济学是主流经济学的一个分支,研究个人和企业在做出有关稀缺资源分配的决策时的行为以及这些个人和企业之间的相互作用。my-assignmentexpert™ 为您的留学生涯保驾护航 在数学Mathematics作业代写方面已经树立了自己的口碑, 保证靠谱, 高质且原创的数学Mathematics代写服务。我们的专家在图论代写Graph Theory代写方面经验极为丰富,各种图论代写Graph Theory相关的作业也就用不着 说。
线性代数代写
线性代数是数学的一个分支,涉及线性方程,如:线性图,如:以及它们在向量空间和通过矩阵的表示。线性代数是几乎所有数学领域的核心。
博弈论代写
现代博弈论始于约翰-冯-诺伊曼(John von Neumann)提出的两人零和博弈中的混合策略均衡的观点及其证明。冯-诺依曼的原始证明使用了关于连续映射到紧凑凸集的布劳威尔定点定理,这成为博弈论和数学经济学的标准方法。在他的论文之后,1944年,他与奥斯卡-莫根斯特恩(Oskar Morgenstern)共同撰写了《游戏和经济行为理论》一书,该书考虑了几个参与者的合作游戏。这本书的第二版提供了预期效用的公理理论,使数理统计学家和经济学家能够处理不确定性下的决策。
微积分代写
微积分,最初被称为无穷小微积分或 “无穷小的微积分”,是对连续变化的数学研究,就像几何学是对形状的研究,而代数是对算术运算的概括研究一样。
它有两个主要分支,微分和积分;微分涉及瞬时变化率和曲线的斜率,而积分涉及数量的累积,以及曲线下或曲线之间的面积。这两个分支通过微积分的基本定理相互联系,它们利用了无限序列和无限级数收敛到一个明确定义的极限的基本概念 。
计量经济学代写
什么是计量经济学?
计量经济学是统计学和数学模型的定量应用,使用数据来发展理论或测试经济学中的现有假设,并根据历史数据预测未来趋势。它对现实世界的数据进行统计试验,然后将结果与被测试的理论进行比较和对比。
根据你是对测试现有理论感兴趣,还是对利用现有数据在这些观察的基础上提出新的假设感兴趣,计量经济学可以细分为两大类:理论和应用。那些经常从事这种实践的人通常被称为计量经济学家。
Matlab代写
MATLAB 是一种用于技术计算的高性能语言。它将计算、可视化和编程集成在一个易于使用的环境中,其中问题和解决方案以熟悉的数学符号表示。典型用途包括:数学和计算算法开发建模、仿真和原型制作数据分析、探索和可视化科学和工程图形应用程序开发,包括图形用户界面构建MATLAB 是一个交互式系统,其基本数据元素是一个不需要维度的数组。这使您可以解决许多技术计算问题,尤其是那些具有矩阵和向量公式的问题,而只需用 C 或 Fortran 等标量非交互式语言编写程序所需的时间的一小部分。MATLAB 名称代表矩阵实验室。MATLAB 最初的编写目的是提供对由 LINPACK 和 EISPACK 项目开发的矩阵软件的轻松访问,这两个项目共同代表了矩阵计算软件的最新技术。MATLAB 经过多年的发展,得到了许多用户的投入。在大学环境中,它是数学、工程和科学入门和高级课程的标准教学工具。在工业领域,MATLAB 是高效研究、开发和分析的首选工具。MATLAB 具有一系列称为工具箱的特定于应用程序的解决方案。对于大多数 MATLAB 用户来说非常重要,工具箱允许您学习和应用专业技术。工具箱是 MATLAB 函数(M 文件)的综合集合,可扩展 MATLAB 环境以解决特定类别的问题。可用工具箱的领域包括信号处理、控制系统、神经网络、模糊逻辑、小波、仿真等。