如果你也在 怎样代写深度学习Deep Learning ACDL2022这个学科遇到相关的难题,请随时右上角联系我们的24/7代写客服。深度学习Deep Learning(也称为深度结构化学习)是更广泛的机器学习方法系列的一部分,它是基于人工神经网络的表征学习。学习可以是监督的、半监督的或无监督的。人工神经网络(ANNs)的灵感来自于生物系统的信息处理和分布式通信节点。人工神经网络与生物大脑有各种不同之处。具体来说,人工神经网络倾向于静态和符号化,而大多数生物体的生物脑是动态(可塑性)和模拟的。
深度学习Deep Learning架构,如深度神经网络、深度信念网络、深度强化学习、递归神经网络、卷积神经网络和变形金刚,已被应用于包括计算机视觉、语音识别、自然语言处理、机器翻译、生物信息学、药物设计、医学图像分析、气候科学、材料检测和棋盘游戏程序等领域,它们产生的结果与人类专家的表现相当,在某些情况下甚至超过了人类专家。
深度学习Deep Learning代写,免费提交作业要求, 满意后付款,成绩80\%以下全额退款,安全省心无顾虑。专业硕 博写手团队,所有订单可靠准时,保证 100% 原创。 最高质量的机器学习Machine Learning作业代写,服务覆盖北美、欧洲、澳洲等 国家。 在代写价格方面,考虑到同学们的经济条件,在保障代写质量的前提下,我们为客户提供最合理的价格。 由于作业种类很多,同时其中的大部分作业在字数上都没有具体要求,因此机器学习Machine Learning作业代写的价格不固定。通常在专家查看完作业要求之后会给出报价。作业难度和截止日期对价格也有很大的影响。
同学们在留学期间,都对各式各样的作业考试很是头疼,如果你无从下手,不如考虑my-assignmentexpert™!
my-assignmentexpert™提供最专业的一站式服务:Essay代写,Dissertation代写,Assignment代写,Paper代写,Proposal代写,Proposal代写,Literature Review代写,Online Course,Exam代考等等。my-assignmentexpert™专注为留学生提供Essay代写服务,拥有各个专业的博硕教师团队帮您代写,免费修改及辅导,保证成果完成的效率和质量。同时有多家检测平台帐号,包括Turnitin高级账户,检测论文不会留痕,写好后检测修改,放心可靠,经得起任何考验!
想知道您作业确定的价格吗? 免费下单以相关学科的专家能了解具体的要求之后在1-3个小时就提出价格。专家的 报价比上列的价格能便宜好几倍。
我们在计算机Quantum computer代写方面已经树立了自己的口碑, 保证靠谱, 高质且原创的计算机Quantum computer代写服务。我们的专家在深度学习Deep Learning代写方面经验极为丰富,各种深度学习Deep Learning相关的作业也就用不着 说。
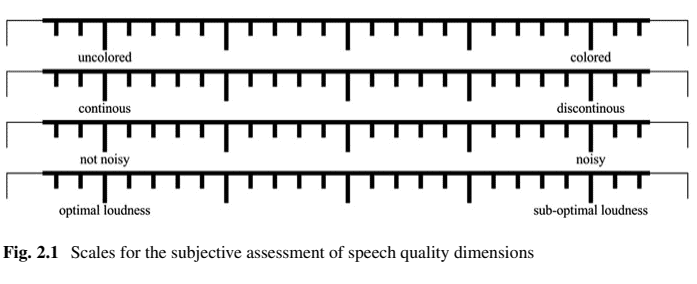
计算机代写|深度学习代写Deep Learning代考|Speech Quality and Speech Quality Dimensions
When speech communication networks are evaluated, one of the main performance indicators is the perceived quality of the transmitted speech. Jekosch (2005) defined speech quality as follows:
Speech quality: The result of assessing all the recognised and nameable features and feature values of a speech sampled under examination, in terms of suitability to fulfil the expectation of all the recognised and nameable features and feature values of individual expectations and/or social demands and/or demands.
Thus, the perceived speech quality is mostly depending on the user’s expectations. When a user assesses the quality of a speech sample, the assessment process is triggered by the physical speech signal. The signal is perceived and reflected by the listener, at which point also the perceived quality features are created within the listener. In parallel to the assessment of the speech sample under examination, a similar process is executed on the listener’s experiences that creates the desired quality features. A comparison and judgement between the desired and perceived quality features then leads to the perceived speech quality.
According to Wältermann (2012), the perceived quality is a multidimensional value and can be thought of as a point in a multidimensional perceptual space. If the coordinate system of this perceptual space is Cartesian and each of the auditory features is orthogonal to each other, the features are referred to as perceptual dimensions. The orthogonality also implies that the values of different perceptual dimensions are not correlated. In this multidimensional space, the listener’s desired composition (internal reference) is defined by a vector $p$ and the perceived composition (speech signal under study) as $\boldsymbol{q}$ such that
$$
\boldsymbol{p}=\left(p_1, \ldots, p_{N_{\mathrm{dim}}}\right), \quad \boldsymbol{q}=\left(q_1, \ldots, q_{N_{\mathrm{dim}}}\right) .
$$
The overall or integral quality $Q$ can then be defined by the Euclidean distance between $\boldsymbol{p}$ and $\boldsymbol{q}$,
$$
Q=d(\boldsymbol{p}, \boldsymbol{q})=\sqrt{\sum_{i=1}^{N_{\mathrm{dim}}} \alpha_i\left(p_i-q_i\right)^2}
$$
where $N_{\mathrm{dim}}$ is the number of orthogonal dimensions and the weighting coefficients $\alpha_i$ determine the influence of each dimension.
计算机代写|深度学习代写Deep Learning代考|Subjective Assessment via Crowdsourcing
Recently the subjective assessment of speech quality through crowdsourcing on platforms such as Amazon Mechanical Turk has attracted increased interest. Although a controlled laboratory setup comes with more measurement reliability, they lack realism as the listening equipment, and the test environment does not reflect the typical usage situation. Furthermore, laboratory experiments are very time-consuming for the experimenters and need to be carefully planned in advance. Micro-task crowdsourcing, on the other hand, offers a fast, low-cost, and scalable approach to collect subjective ratings from a geographically distributed pool of demographically diverse participants that use a wide range of listening devices.
In crowdsourcing subjective tests, the assessment task is provided to the crowdworkers over the Internet. The participating crowdworkers are usually remunerated for their work. Recently, an ITU-T Recommendation for conducting speech quality tests via crowdsourcing has been published as ITU-T Rec. P.808 (2018). The Recommendation describes the experiment design, test procedure, and data analyses for an ACR listening-only speech quality test. Furthermore, it contains methods to evaluate the participant’s test eligibility (i.e. hearing test), environment/listening system suitability, and quality control mechanisms. A recent study by Naderi et al. (2020) shows that valid and reliable results for speech quality assessment in crowdsourcing are achieved if the tests are conducted according to ITU-T Rec. P.808 (2018).
The following points should be considered when tests in the crowd are conducted:
Source material The preparation of the source material is the same as for a lab test according to ITU-T Rec. P.808.
Test duration As typical crowdsourcing micro-tasks only take a few minutes to complete, it is recommended to split an experiment into a chain of these smaller tasks, where one task should contain 5-15 stimuli. However, a crowdworker may only perform one of these tasks and as a result does not rate the entire set of stimuli available. This lack of context can lead to an error variance in the ratings caused by the corpus effect. To overcome this problem, individual tasks with $5-15$ samples can be prepared to contain a good variety of conditions. Also, the crowdworkers can be motivated to complete more tasks by offering an extra reward (i.e. bonus) when a certain minimum number of tasks are completed.
Test procedure It is recommended to create overall three jobs for the crowdsourcing assessment: qualification job, training job, and rating job.
Qualification job In the qualification job, the purpose of the study is explained to the crowdworkers, and it is checked whether they are eligible to participate. For example, it could be asked if they suffer from hearing loss. Also, to guarantee a balanced participant distribution, the participants can be asked for their gender and age. Based on the response, a randomly sampled group of crowdworkers (who satisfied the prerequisites) can be invited to participate in the experiment. Instead of using a qualification job (or additionally), also, in-built platform features can be used, such as only inviting crowdworkers with a task approval rate of at least $98 \%$ and a minimum of 500 approved tasks.
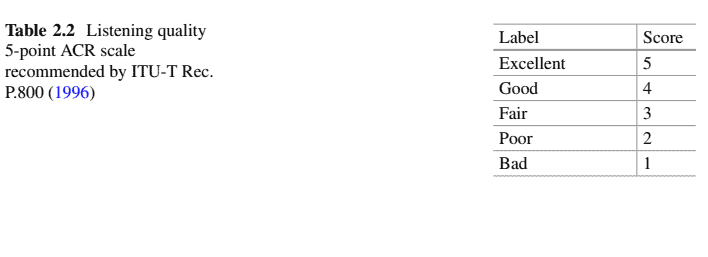
深度学习代写
计算机代写深度学习代写DEEP LEARNING代考|SPEECH QUALITY AND SPEECH QUALITY DIMENSIONS
在评估语音通信网络时,主要性能指标之一是传输语音的感知质量。耶科施 2005 定义语音质量如下:
语音质量:评估在检音中采样的语音的所有可识别和可命名的特征和特征值的结果,在满足个人期望和/或社会需求的所有可识别和可命名的特征和特征值的期肓 方面的适用性和/或社会需求和/或要求。
因此,感知的语音质量主要取决于用户的期望。当用户评估语音样本的质量时,评估过程由物理语音信昊触发。信号被收听者感知和反射,此时在收听者内部也创 建了感知质量特征。在评估被检查的语音样本的同时,对听众的体验执行类似的过程,以命建所需的质量特征。期望和感知质量特征之间的比较和判断然后导致感 知语音质亘。
根据沃尔特夢 2012 ,感知质量是一个多维值,可以认为是多维感知空间中的一个点。如果这个咸知空间的坐标系是笛卡尔坐标系并且每个听觉特征相互正交,则 这些特征被称为感知维度。正交性还意味着不同感知维度的值不相关。在这个侈维空间中,听者想要的构图internalreference由向量定义p和感知的成分 speechsignalunderstudy作为 $q$ 这样
$$
\boldsymbol{p}=\left(p_1, \ldots, p_{N_{\text {diim }}}\right), \quad \boldsymbol{q}=\left(q_1, \ldots, q_{N_{\mathrm{dim}}}\right)
$$
整体或整体质量 $Q$ 然后可以由之间的欧几里得距离定义 $p$ 和 $q$,
$$
Q=d(\boldsymbol{p}, \boldsymbol{q})=\sqrt{\sum_{i=1}^{N_{\mathrm{dim}}} \alpha_i\left(p_i-q_i\right)^2}
$$
在哪里 $N_{\mathrm{dim}}$ 是正交维数和加权䒩数 $\alpha_i$ 确定每个维度的影响。
计算机代写深度学习代写DEEP LEARNING代考|SUBJECTIVE ASSESSMENT VIA CROWDSOURCING
最近,通过 Amazon Mechanical Turk等平台上的众包对语音质量的主观评估引起了越来越多的兴趣。虽然受控的实验室设置具有更高的测量可靠性,但它们作为听 速、低成本和可扩展的方法,可以从使用各种收听设备的地理分布不同的参与者池中收集主观评分。
在众包主观测试中,评估任务通过互联网提供给众包工作者。参与的众包工作者通常因其工作而获得报酬。最近,通过众包进行语音质量测试的 ITU-T建议书已发 布为 ITU-T Rec. P.8082018. 该建议书描述了 ACR只听语音质量测试的实验设计、则试程序和数据分析。此外,它还包含评估参与者测试秶格的方法
i.e. hearingtest、环境/听力系统适用性和质量控制机制。Naderi 等人最近的一项研究。2020表明如果根据 ITU-T Rec. 进行则试,则可以在众包中获得有效和可靠 的语音质量评估结果。P.8082018.
在人群中进行财试时应考虑以下几点:
源材料 源材料的准备与根据 ITU-T Rec. 进行的实验室测试相同。第 808 页。
测试持续时间 由于典型的众包微任务只需几分钟即可完成,因此建议将实验拆分为一系列较小的任务,其中一个任务应包含 5-15个刺激。但是,众包工作者可能 只执行其中一项任务,因此不会对可用的整个刺激集进行评分。这种缺工上下文可能导致由语料库效应引起的评级误差差异。为了克服这个问题,单独的任务与 $5-15$ 样品可以制䔰成包含多种条件。此外,可以通过提供额外奖厉来激励众包工作者完成更㝖任务i. $i . b o n u s$ 当完成某个最小数量的任务时。
财试程序众包评估建议创建三个工作:资格工作、培训工作和评级工作。
资格工作在资格工作中,向众包工作人员解释研究目的,并检龺他们是否有资格参加。例如,可以询问他们是否患有听力损失。此外,为了保证参与者分布的平 衡,可以询问参与者的性别和年龄。根据响应,随机抽样的人群工作组whosatisfiedtheprerequisites 可以被邀请参加实验。而不是使用资格认证工作 oradditionally,也可以使用平台内置功能,比如只邀请任务批准率不低于 $98 \%$ 以及至少 500 个已批准的任务。
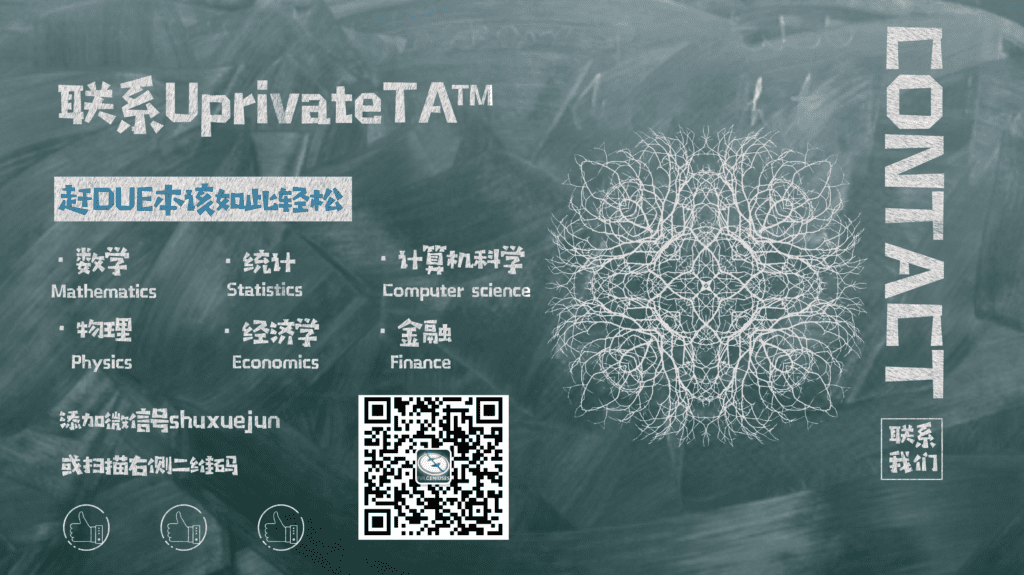
计算机代写|深度学习代写Deep Learning代考 请认准UprivateTA™. UprivateTA™为您的留学生涯保驾护航。
微观经济学代写
微观经济学是主流经济学的一个分支,研究个人和企业在做出有关稀缺资源分配的决策时的行为以及这些个人和企业之间的相互作用。my-assignmentexpert™ 为您的留学生涯保驾护航 在数学Mathematics作业代写方面已经树立了自己的口碑, 保证靠谱, 高质且原创的数学Mathematics代写服务。我们的专家在图论代写Graph Theory代写方面经验极为丰富,各种图论代写Graph Theory相关的作业也就用不着 说。
线性代数代写
线性代数是数学的一个分支,涉及线性方程,如:线性图,如:以及它们在向量空间和通过矩阵的表示。线性代数是几乎所有数学领域的核心。
博弈论代写
现代博弈论始于约翰-冯-诺伊曼(John von Neumann)提出的两人零和博弈中的混合策略均衡的观点及其证明。冯-诺依曼的原始证明使用了关于连续映射到紧凑凸集的布劳威尔定点定理,这成为博弈论和数学经济学的标准方法。在他的论文之后,1944年,他与奥斯卡-莫根斯特恩(Oskar Morgenstern)共同撰写了《游戏和经济行为理论》一书,该书考虑了几个参与者的合作游戏。这本书的第二版提供了预期效用的公理理论,使数理统计学家和经济学家能够处理不确定性下的决策。
微积分代写
微积分,最初被称为无穷小微积分或 “无穷小的微积分”,是对连续变化的数学研究,就像几何学是对形状的研究,而代数是对算术运算的概括研究一样。
它有两个主要分支,微分和积分;微分涉及瞬时变化率和曲线的斜率,而积分涉及数量的累积,以及曲线下或曲线之间的面积。这两个分支通过微积分的基本定理相互联系,它们利用了无限序列和无限级数收敛到一个明确定义的极限的基本概念 。
计量经济学代写
什么是计量经济学?
计量经济学是统计学和数学模型的定量应用,使用数据来发展理论或测试经济学中的现有假设,并根据历史数据预测未来趋势。它对现实世界的数据进行统计试验,然后将结果与被测试的理论进行比较和对比。
根据你是对测试现有理论感兴趣,还是对利用现有数据在这些观察的基础上提出新的假设感兴趣,计量经济学可以细分为两大类:理论和应用。那些经常从事这种实践的人通常被称为计量经济学家。
Matlab代写
MATLAB 是一种用于技术计算的高性能语言。它将计算、可视化和编程集成在一个易于使用的环境中,其中问题和解决方案以熟悉的数学符号表示。典型用途包括:数学和计算算法开发建模、仿真和原型制作数据分析、探索和可视化科学和工程图形应用程序开发,包括图形用户界面构建MATLAB 是一个交互式系统,其基本数据元素是一个不需要维度的数组。这使您可以解决许多技术计算问题,尤其是那些具有矩阵和向量公式的问题,而只需用 C 或 Fortran 等标量非交互式语言编写程序所需的时间的一小部分。MATLAB 名称代表矩阵实验室。MATLAB 最初的编写目的是提供对由 LINPACK 和 EISPACK 项目开发的矩阵软件的轻松访问,这两个项目共同代表了矩阵计算软件的最新技术。MATLAB 经过多年的发展,得到了许多用户的投入。在大学环境中,它是数学、工程和科学入门和高级课程的标准教学工具。在工业领域,MATLAB 是高效研究、开发和分析的首选工具。MATLAB 具有一系列称为工具箱的特定于应用程序的解决方案。对于大多数 MATLAB 用户来说非常重要,工具箱允许您学习和应用专业技术。工具箱是 MATLAB 函数(M 文件)的综合集合,可扩展 MATLAB 环境以解决特定类别的问题。可用工具箱的领域包括信号处理、控制系统、神经网络、模糊逻辑、小波、仿真等。