如果你也在 怎样代写随机分析stochastic analysis MA547这个学科遇到相关的难题,请随时右上角联系我们的24/7代写客服。随机分析stochastic analysis或随机过程可以被定义为由一些数学集合索引的随机变量的集合,这意味着随机过程的每个随机变量都与该集合中的一个元素唯一相关。历史上,索引集是实线的某个子集,如自然数,从而使索引集有了时间的解释。集合中的每个随机变量都从同一数学空间取值,称为状态空间。
随机分析stochastic analysis在概率论和相关领域,随机(/stoʊˈkæstɪk/)或随机过程是一个数学对象,通常被定义为一个随机变量系列。随机过程被广泛用作系统和现象的数学模型,这些系统和现象似乎以随机的方式变化。这方面的例子包括细菌种群的生长,由于热噪声而波动的电流,或气体分子的运动。 随机过程在许多学科中都有应用,如生物学、化学、生态学、神经科学、物理学、 图像处理、信号处理、控制理论、信息理论、计算机科学、密码学和电信。此外,金融市场中看似随机的变化也促使随机过程在金融中得到广泛使用。
随机分析stochastic analysis代写,免费提交作业要求, 满意后付款,成绩80\%以下全额退款,安全省心无顾虑。专业硕 博写手团队,所有订单可靠准时,保证 100% 原创。最高质量的随机分析stochastic analysis作业代写,服务覆盖北美、欧洲、澳洲等 国家。 在代写价格方面,考虑到同学们的经济条件,在保障代写质量的前提下,我们为客户提供最合理的价格。 由于统计Statistics作业种类很多,同时其中的大部分作业在字数上都没有具体要求,因此随机分析stochastic analysis作业代写的价格不固定。通常在经济学专家查看完作业要求之后会给出报价。作业难度和截止日期对价格也有很大的影响。
同学们在留学期间,都对各式各样的作业考试很是头疼,如果你无从下手,不如考虑my-assignmentexpert™!
my-assignmentexpert™提供最专业的一站式服务:Essay代写,Dissertation代写,Assignment代写,Paper代写,Proposal代写,Proposal代写,Literature Review代写,Online Course,Exam代考等等。my-assignmentexpert™专注为留学生提供Essay代写服务,拥有各个专业的博硕教师团队帮您代写,免费修改及辅导,保证成果完成的效率和质量。同时有多家检测平台帐号,包括Turnitin高级账户,检测论文不会留痕,写好后检测修改,放心可靠,经得起任何考验!
想知道您作业确定的价格吗? 免费下单以相关学科的专家能了解具体的要求之后在1-3个小时就提出价格。专家的 报价比上列的价格能便宜好几倍。
我们在金融 Finaunce代写方面已经树立了自己的口碑, 保证靠谱, 高质且原创的金融 Finaunce代写服务。我们的专家在随机分析stochastic analysis代写方面经验极为丰富,各种随机分析stochastic analysis相关的作业也就用不着 说。
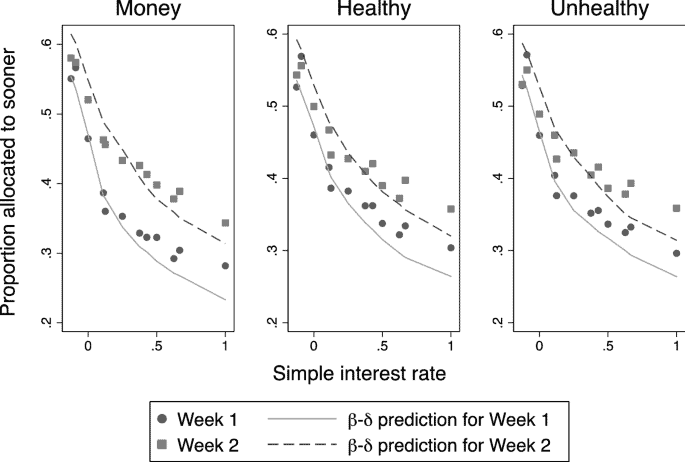
数学代写|随机分析代写Stochastic Calculus代考|Present-bias Preferences
Present-biased preferences, also known as hyperbolic discounting, refer to the following observation in intertemporal choice: when considering time preferences between two moments, individuals become more impatient when the two moments are closer to the present time. Thaler (1981) provides an illustrative example of presentbiased preferences: some people may prefer an apple today to two apples tomorrow, but very few people would prefer an apple in a year to two apples in a year plus one day. Noted as early as in Strotz (1955-1956), present-biased preferences lead to time inconsistency. For example, consider an agent whose time preferences for having apples are as described in the above illustrative example by Thaler (1981). At time 0, faced with Option A of having one apple at time $t=365$ (days) and Option B of having two apples at time $s=366$ (days), the agent chooses Option B. When time $t=365$ arrives, however, if the agent gets to choose again, she would choose Option A. This shows that the agent in the future will change her actions planned today; hence time-inconsistency is present. For a review of the literature on present-biased preferences, see Frederick et al. (2002).
In a time-separable discounted utility model, present-biased preferences can be modeled by a non-exponential discount function. For example, consider an intertemporal consumption model in continuous time for an agent. The agent’s preference value of a random consumption stream $\left(C_s\right)_{s \in[t, T]}$ can be represented as
$$
\mathbb{E}_t\left[\int_t^T h(s-t) u\left(C_s\right) d s\right],
$$
where $u$ is the agent’s utility function, $h$ is the agent’s discount function, and $\mathbb{E}_t$ denotes the expectation conditional on all the information available at time $t$. To model present-biased preferences, we assume $h(s+\Delta) / h(s)$ to be strictly increasing in $s \geq 0$ for any fixed $\Delta>0$; hence it excludes the standard exponential discount function. An example is the generalized hyperbolic discount function proposed by Loewenstein and Prelec (1992): $h(s)=(1+\alpha s)^{-\beta / \alpha}, s \geq 0$, where $\alpha>0$ and $\beta>$ 0 are two parameters. Ebert et al. (2020) introduce a class of weighted discount functions that is broad enough to include most commonly used non-exponential discount functions in finance and economics.
In various continuous-time settings, Barro (1999), Ekeland and Lazrak (2006), Ekeland and Lazrak (2008), Ekeland and Lazrak (2010), Ekeland and Pirvu (2008), Marín-Solano and Navas (2010), and Ekeland et al. (2012) study intra-personal equilibria for portfolio selection and consumption problems with present-biased preferences. Ebert et al. (2020) and Tan et al. (2018) study real option problems for agents with general weighted discount functions and derive equilibrium investment strategies. Harris and Laibson (2013) and Grenadier and Wang (2007) apply a stochastic, piece-wise step discount function to a consumption problem and a real option problem, respectively, and derive intra-personal equilibrium strategies. Asset pricing for sophisticated agents with present-biased preferences and without commitment has been studied by Luttmer and Mariotti (2003) and Björk et al. (2017).
数学代写|随机分析代写Stochastic Calculus代考|Mean-Variance
A popular decision criterion in finance is mean-variance, with which an agent minimizes the variance and maximizes the mean of certain random quantity, e.g., the wealth of a portfolio at the end of a period. Any mean-variance model is inherently time inconsistent due to the variance part. To see this, consider a two-period decision problem with dates 0,1 , and 2 for an agent. The agent is offered various options at time 1 that will yield certain payoffs at time 2. The set of options offered to the agent at time 1 depends on the outcome of a fair coin that is tossed between time 0 and 1. If the toss yields a head, the agent is offered two options at time 1: Option H1 that yields $\$ 0$ and $\$ 200$ with equal probabilities and Option $\mathrm{H} 2$ that yields $\$ 50$ and $\$ 150$ with equal probabilities. If the toss yields a tail, the agent is offered another two options at time 1: Option T1 that yields $\$ 0$ and $\$ 200$ with equal probabilities and Option T2 that yields $\$ 1050$ and $\$ 1150$ with equal probabilities. Suppose that at both time 0 and 1 , the agent’s decision criterion is to minimize the variance of the terminal payoff at time 2. At time 0 , the agent has not yet observed the outcome of the toss; so she will need to make choices contingent on this outcome, i.e., she chooses between the following four plans: $(\mathrm{H} 1, \mathrm{~T} 1),(\mathrm{H} 1, \mathrm{~T} 2),(\mathrm{H} 2, \mathrm{~T} 1)$, and $(\mathrm{H} 2, \mathrm{~T} 2)$, where the first and second components of each of the above four plans stand for the agent’s planned choice when the toss yields a head and a tail, respectively. Straightforward calculation shows that the plan ( $\mathrm{H} 2, \mathrm{~T} 1)$ yields the smallest variance of the terminal payoff; so at time 0 the agent plans to choose $\mathrm{H} 2$ when the toss yields a head and choose T1 when the toss yields a tail. At time 1 , after having observed the outcome of the toss, if the agent can choose again with the objective of minimizing the variance of the terminal payoff, she would choose $\mathrm{H} 2$ if the outcome is a head and T2 is the outcome is a tail. Consequently, what the agent plans at time 0 is different from what is optimal for the agent at time 1, resulting in time inconsistency.
The reason of having time inconsistency above can be seen from the following conditional variance formula: $\operatorname{var}(X)=\mathbb{E}[\operatorname{var}(X \mid Y)]+\operatorname{var}(\mathbb{E}[X \mid Y])$, where $X$ stands for the terminal payoff and $Y$ denotes the outcome of the coin toss. At time 0 , the agent’s objective is to $\operatorname{maximize} \operatorname{var}(X)$ and at time 1 , her objective is to maxi$\operatorname{mize} \operatorname{var}(X \mid Y)$. Although the plan (H2,T2) yields small variance of $X$ given the outcome of the toss $Y$ and thus a small value of the average conditional variance $\mathbb{E}[\operatorname{var}(X \mid Y)]$, it yields very different expected payoffs conditional on having a head and on having a tail, leading to a large value of $\operatorname{var}(\mathbb{E}[X \mid Y])$. Consequently, $\operatorname{var}(X)$ under plan $(\mathrm{H} 2, \mathrm{~T} 2)$ is larger than under plan $(\mathrm{H} 2, \mathrm{~T} 1)$, which yields a larger value of $\mathbb{E}[\operatorname{var}(X \mid Y)]$ than the former but a much smaller value of $\operatorname{var}(\mathbb{E}[X \mid Y])$. Consequently, $(\mathrm{H} 2, \mathrm{~T} 1)$ is preferred to $(\mathrm{H} 2, \mathrm{~T} 2)$ for the agent at time 0 .
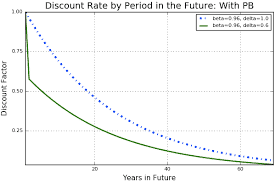
随机分析代写
数学代写|随机分析代寻STOCHASTIC CALCULUS代考|PRESENT-BIAS PREFERENCES
现在偏向偏好,也称为双曲线贴现,指的是跨期选择中的以下观察:在考虑两个时刻之间的时间偏好时,当两个时刻更接近现在时,个体变得更加不耐烦。泰勒 1981提供了一个说明性偏好的例子:有些人今天可能更喜欢一个苹果而不是明天两个苹果,但是很少有人会更喜欢一年后的一个苹果而不是一年加一天后的两个 苹果。早在 Strotz 就注意到了1955-1956,现在偏见的偏好导致时间不一致。例如,考虑一个代理人,其对苹果的时间偏好如上述 Thaler 的说明性示例中所述 1981. 在 0 时刻,面临选择 $\mathrm{A}$ 的时刻有一个苹果 $t=365$ days 和一次有两个苹果的选项 $\mathrm{B} s=366$ days,代理人选择选项 $\mathrm{B}$ 。当时间 $t=365$ 然而,如果代理人再次 选择,她会选择选项 A。这表明末来的代理人将改变她今天计划的行动;因此存在时间不一致。有关当前偏好的文献综述,请参阅 Frederick 等人。2002.
在时间可分贴现效用模型中,当前偏向偏好可以通过非指数贴现函数建模。例如,考虑代理人在连续时间内的跨期消费模型。代理对随机消费流的偏好值 $\left(C_s\right)_{s \in[t, T]}$ 可以表示为
$$
\mathbb{E}_t\left[\int_t^T h(s-t) u\left(C_s\right) d s\right],
$$
在哪里 $u$ 是代理人的效用函数, $h$ 是代理人的折扣函数,并且 $\mathbb{E}_t$ 表示以当时所有可用信息为条件的期望 $t$. 为了模拟现在偏向的偏好,我们假设 $h(s+\Delta) / h(s)$ 严格 递增 $s \geq 0$ 对于任何固定 $\Delta>0$; 因此它排除了标准的指数折扣函数。一个例子是 Loewenstein 和 Prelec 提出的广义双曲贴现函数 1992:
$h(s)=(1+\alpha s)^{-\beta / \alpha}, s \geq 0$ ,在哪里 $\alpha>0$ 和 $\beta>0$ 是两个参数。埃伯特等人。2020引入一类加权贴现函数,其范围足以包括金融和经济学中最常用的非指数贴 现函数。
在各种连续时间设置中,Barro1999, Ekeland 和 Lazrak2006, Ekeland 和 Lazrak2008, Ekeland 和 Lazrak2010, Ekeland 和 Pirvu2008、马林-索拉诺和纳瓦斯2010和 Ekeland 等人。2012研究投资组合选择和消费问题的个人内部均衡与当前偏好。埃伯特等人。2020和 Tan 等人。2018研究具有一般加权贴现函数的代理人的实物 期权问题并得出均衡投资策略。哈里斯和莱布森2013和郑弹兵和王2007分别对消费问题和实物期权问题应用随机的、分段的贴现函数,并得出个人内部均衡策 略。Luttmer 和 Mariotti 研究了具有当前偏好且没有承诺的复杂代理的资产定价2003和比约克等人。2017.
数学代写|随机分析代写STOCHASTIC CALCULUS代考|MEANVARIANCE
金融中一个流行的决策标准是均值-方差,代理人使用它最小化方差并最大化某个随机量的均值,例如,一个时期结束时投资组合的财富。由于方差部分,任何均 值-方差模型本质上都是时间不一致的。要看到这一点,请考虑代理人的日期为 0,1 和 2 的两期决策问题。在时间 1 向代理人提供各种选项,这些选项将在时间 2 产 生一定的收益。在时间 1 提供给代理人的选项集取决于在时间 0 和 1 之间扐郑的公平硬币的结果。如果抛烪产生a head, agent 在时间 1 有两个选项: 选项 H1产生 $\$ 0$ 和 $\$ 200$ 具有相同的概率和选项 $\mathrm{H} 2$ 产生 $\$ 50$ 和 $\$ 150$ 以相等的概率。如果抛䣋结果为尾巴,则在时间 1 时向代理人提供另外两个选项: 选项 T1 产生 $\$ 0$ 和 $\$ 200$ 具有相 同的概率和选项 T2产生 $\$ 1050$ 和 $\$ 1150$ 以相等的概率。假设在时间 0 和 1 , agent 的决策准则都是最小化时间 2 的终端收益的方差。在时间 0 , agent 还没有观察到 抛郱的结果;所以她需要根据这个结果做出选择,即她在以下四个计划中做出选择:(H1, T1), (H1, T2), (H2, T1),和(H2,T2),其中上述四个计划中的每个的第一和第二部分分别代表当抛郑产生正面和反面时代理的计划选择。简单的计算表明,该计划 $\$ H 2, T 1$
yieldsthesmallestvarianceoftheterminalpayoff; soattime 0 theagentplanstochoose $\backslash \operatorname{mat} \mathrm{hr} \mathrm{m}[\mathrm{H}} 2$
whenthetossyieldsaheadandchooseT1 whenthetossyieldsatail. Attime1, afterhavingobservedtheoutcomeofthetoss, iftheagentcanchooseagainwitht $\backslash \operatorname{mathrm}{\mathrm{H}} 2$
iftheoutcomeisaheadandT2istheoutcomeisatail. Consequently, whattheagentplansattime0isdifferent fromwhatisoptimalfortheagentattime1, res
|运营商名称 $[$ 在哪里 $} X={\operatorname{mathbb}[\mathrm{E}}$
$$
\operatorname{var}(X \mid Y)
$$
$+1$ 运荣商名称[哪里 $] \mathbb{E}[X \mid Y]$, whereXstands fortheterminalpayof fand 是 denotestheoutcomeof thecointoss. Attime 0 , theagent’sobjectiveisto
. Althoughtheplan $(H 2, T 2)$ yieldssmallvarianceof $\times$ giventheoutcomeofthetoss 是 andthusasmallvalueoftheaverageconditionalvariance $\backslash$ mathbb ${E}$
$$
\operatorname{var}(X \mid Y)
$$
, ityieldsverydifferentexpectedpayoffsconditionalonhavingaheadandonhavingatail, leadingtoalargevalueof $\mid$ 运菅商名称 ${$ 在哪里 $] \mathbb{E}[X \mid Y]$
. Consequently, $\mid$ 运荌商名称 ${$ 在哪里 $}$ Xunderplan $\mathrm{H} 2$, T2islargerthanunderplan $\mathrm{H} 2$, T1, whichyieldsalargervalueof $\backslash \mathrm{mathb} \mathrm{b}[\mathrm{E}}$
$$
\operatorname{var}(X \mid Y)
$$
thanthe formerbutamuchsmallervalueof 运菅商名称 $[$ 在哪里 $] \mathbb{E}[X \mid Y]$. Consequently, $\mathrm{H} 2$, T1ispreferredto $\mathrm{H} 2$, T2\$ 代理人在时间 0 。
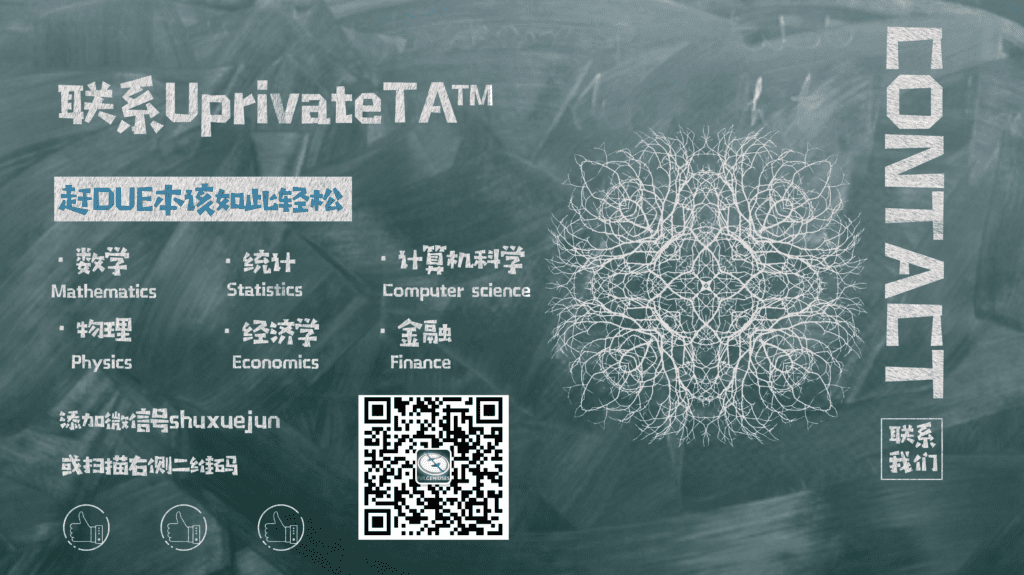
数学代写|随机分析代写Stochastic Analysis in Finance代考 请认准UprivateTA™. UprivateTA™为您的留学生涯保驾护航。
微观经济学代写
微观经济学是主流经济学的一个分支,研究个人和企业在做出有关稀缺资源分配的决策时的行为以及这些个人和企业之间的相互作用。my-assignmentexpert™ 为您的留学生涯保驾护航 在数学Mathematics作业代写方面已经树立了自己的口碑, 保证靠谱, 高质且原创的数学Mathematics代写服务。我们的专家在图论代写Graph Theory代写方面经验极为丰富,各种图论代写Graph Theory相关的作业也就用不着 说。
线性代数代写
线性代数是数学的一个分支,涉及线性方程,如:线性图,如:以及它们在向量空间和通过矩阵的表示。线性代数是几乎所有数学领域的核心。
博弈论代写
现代博弈论始于约翰-冯-诺伊曼(John von Neumann)提出的两人零和博弈中的混合策略均衡的观点及其证明。冯-诺依曼的原始证明使用了关于连续映射到紧凑凸集的布劳威尔定点定理,这成为博弈论和数学经济学的标准方法。在他的论文之后,1944年,他与奥斯卡-莫根斯特恩(Oskar Morgenstern)共同撰写了《游戏和经济行为理论》一书,该书考虑了几个参与者的合作游戏。这本书的第二版提供了预期效用的公理理论,使数理统计学家和经济学家能够处理不确定性下的决策。
微积分代写
微积分,最初被称为无穷小微积分或 “无穷小的微积分”,是对连续变化的数学研究,就像几何学是对形状的研究,而代数是对算术运算的概括研究一样。
它有两个主要分支,微分和积分;微分涉及瞬时变化率和曲线的斜率,而积分涉及数量的累积,以及曲线下或曲线之间的面积。这两个分支通过微积分的基本定理相互联系,它们利用了无限序列和无限级数收敛到一个明确定义的极限的基本概念 。
计量经济学代写
什么是计量经济学?
计量经济学是统计学和数学模型的定量应用,使用数据来发展理论或测试经济学中的现有假设,并根据历史数据预测未来趋势。它对现实世界的数据进行统计试验,然后将结果与被测试的理论进行比较和对比。
根据你是对测试现有理论感兴趣,还是对利用现有数据在这些观察的基础上提出新的假设感兴趣,计量经济学可以细分为两大类:理论和应用。那些经常从事这种实践的人通常被称为计量经济学家。
MATLAB代写
MATLAB 是一种用于技术计算的高性能语言。它将计算、可视化和编程集成在一个易于使用的环境中,其中问题和解决方案以熟悉的数学符号表示。典型用途包括:数学和计算算法开发建模、仿真和原型制作数据分析、探索和可视化科学和工程图形应用程序开发,包括图形用户界面构建MATLAB 是一个交互式系统,其基本数据元素是一个不需要维度的数组。这使您可以解决许多技术计算问题,尤其是那些具有矩阵和向量公式的问题,而只需用 C 或 Fortran 等标量非交互式语言编写程序所需的时间的一小部分。MATLAB 名称代表矩阵实验室。MATLAB 最初的编写目的是提供对由 LINPACK 和 EISPACK 项目开发的矩阵软件的轻松访问,这两个项目共同代表了矩阵计算软件的最新技术。MATLAB 经过多年的发展,得到了许多用户的投入。在大学环境中,它是数学、工程和科学入门和高级课程的标准教学工具。在工业领域,MATLAB 是高效研究、开发和分析的首选工具。MATLAB 具有一系列称为工具箱的特定于应用程序的解决方案。对于大多数 MATLAB 用户来说非常重要,工具箱允许您学习和应用专业技术。工具箱是 MATLAB 函数(M 文件)的综合集合,可扩展 MATLAB 环境以解决特定类别的问题。可用工具箱的领域包括信号处理、控制系统、神经网络、模糊逻辑、小波、仿真等。