如果你也在 怎样代写贝叶斯分析Bayesian Analysis 这个学科遇到相关的难题,请随时右上角联系我们的24/7代写客服。贝叶斯分析Bayesian Analysis一种统计推断方法(以英国数学家托马斯-贝叶斯命名),它允许人们将关于人口参数的先验信息与样本中包含的信息证据相结合,以指导统计推断过程。首先指定一个感兴趣的参数的先验概率分布。然后通过应用贝叶斯定理获得并结合证据,为参数提供一个后验概率分布。后验分布为有关该参数的统计推断提供了基础。
贝叶斯分析Bayesian Analysis自1763年以来,我们现在所知道的贝叶斯统计学并没有一个明确的运行。尽管贝叶斯的方法被拉普拉斯和当时其他领先的概率论者热情地接受,但在19世纪却陷入了不光彩的境地,因为他们还不知道如何正确处理先验概率。20世纪上半叶,一种完全不同的理论得到了发展,现在称为频繁主义统计学。但贝叶斯思想的火焰被少数思想家保持着,如意大利的布鲁诺-德-菲内蒂和英国的哈罗德-杰弗里斯。现代贝叶斯运动开始于20世纪下半叶,由美国的Jimmy Savage和英国的Dennis Lindley带头,但贝叶斯推断仍然极难实现,直到20世纪80年代末和90年代初,强大的计算机开始广泛使用,新的计算方法被开发出来。随后,人们对贝叶斯统计的兴趣大增,不仅导致了贝叶斯方法论的广泛研究,也导致了使用贝叶斯方法来解决天体物理学、天气预报、医疗保健政策和刑事司法等不同应用领域的迫切问题。
同学们在留学期间,都对各式各样的作业考试很是头疼,如果你无从下手,不如考虑my-assignmentexpert™!
my-assignmentexpert™提供最专业的一站式服务:Essay代写,Dissertation代写,Assignment代写,Paper代写,Proposal代写,Proposal代写,Literature Review代写,Online Course,Exam代考等等。my-assignmentexpert™专注为留学生提供Essay代写服务,拥有各个专业的博硕教师团队帮您代写,免费修改及辅导,保证成果完成的效率和质量。同时有多家检测平台帐号,包括Turnitin高级账户,检测论文不会留痕,写好后检测修改,放心可靠,经得起任何考验!
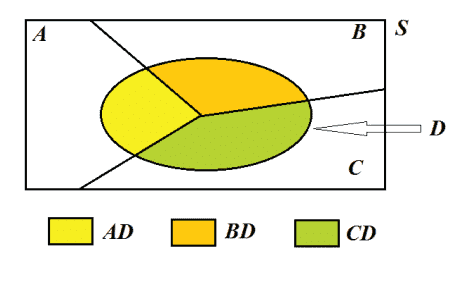
统计代写|贝叶斯分析代考Bayesian Analysis代写|Bayesian models
Bayes’ formula extends naturally to statistical models. A Bayesian model is a parametric model in the classical (or frequentist) sense, but with the addition of a prior probability distribution for the model parameter, which is treated as a random variable rather than an unknown constant. The basic components of a Bayesian model may be listed as:
the data, denoted by $y$
the parameter, denoted by $\theta$
the model distribution, given by a specification of $f(y \mid \theta)$ or $F(y \mid \theta)$ or the distribution of $(y \mid \theta)$
the prior distribution, given by a specification of $f(\theta)$ or $F(\theta)$ or the distribution of $\theta$.
Here, $F$ is a generic symbol which denotes cumulative distribution function (cdf), and $f$ is a generic symbol which denotes probability density function (pdf) (when applied to a continuous random variable) or probability mass function $(\mathrm{pmf})$ (when applied to a discrete random variable). For simplicity, we will avoid the term pmf and use the term pdf or density for all types of random variable, including the mixed type.
Note 1: A mixed distribution is defined by a cdf which exhibits at least one discontinuity (or jump) and is strictly increasing over at least one interval of values.
Note 2: The prior may be specified by writing a statement of the form ‘ $\theta \sim \ldots$, , where the symbol ‘ $\sim$ ‘ means ‘is distributed as’, and where ‘…’denotes the relevant distribution. Likewise, the model for the data may be specified by writing a statement of the form ‘ $(y \mid \theta) \sim \ldots$..
Note 3: At this stage we will not usually distinguish between $y$ as a random variable and $y$ as a value of that random variable; but sometimes we may use $Y$ for the former. Each of $y$ and $\theta$ may be a scalar, vector, matrix or array. Also, each component of $y$ and $\theta$ may have a discrete distribution, a continuous distribution, or a mixed distribution.
In the first few examples below, we will focus on the simplest case where both $y$ and $\theta$ are scalar and discrete.
统计代写|贝叶斯分析代考Bayesian Analysis代写|The posterior distribution
Bayesian inference requires determination of the posterior probability distribution of $\theta$. This task is equivalent to finding the posterior pdf of $\theta$, which may be done using the equation
$$
f(\theta \mid y)=\frac{f(\theta) f(y \mid \theta)}{f(y)}
$$
Here, $f(y)$ is the unconditional (or prior) pdf of $y$, as given by
$$
f(y)=\int f(y \mid \theta) d F(\theta)= \begin{cases}\int f(\theta) f(y \mid \theta) d \theta & \text { if } \theta \text { is continuous } \ \sum_\theta f(\theta) f(y \mid \theta) & \text { if } \theta \text { is discrete. }\end{cases}
$$
Note: Here, $\int f(y \mid \theta) d F(\theta)$ is a Lebesgue-Stieltjes integral, which may need evaluating by breaking the integral into two parts in the case where $\theta$ has a mixed distribution. In the continuous case, think of $d F(\theta)$ as $\frac{d F(\theta)}{d \theta} d \theta=f(\theta) d \theta$
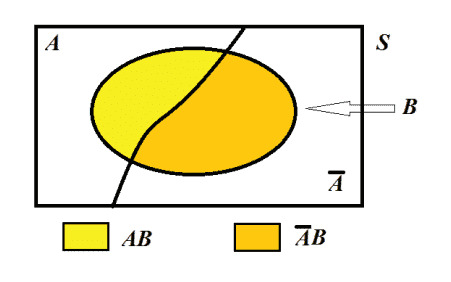
贝叶斯分析代写
统计代写|贝叶斯分析代考Bayesian Analysis代写|Bayesian models
贝叶斯公式自然地扩展到统计模型。贝叶斯模型是经典(或频率论)意义上的参数模型,但增加了模型参数的先验概率分布,该模型参数被视为随机变量而不是未知常数。贝叶斯模型的基本组成部分可以列出如下:
数据用$y$表示
参数,用$\theta$表示
模型分布,由$f(y \mid \theta)$或$F(y \mid \theta)$的规范或$(y \mid \theta)$的分布给出
先验分布,由$f(\theta)$或$F(\theta)$的规范或$\theta$的分布给出。
这里,$F$是表示累积分布函数(cdf)的通用符号,$f$是表示概率密度函数(pdf)(当应用于连续随机变量时)或概率质量函数$(\mathrm{pmf})$(当应用于离散随机变量时)的通用符号。为简单起见,我们将避免使用术语pmf,而对所有类型的随机变量(包括混合类型)使用术语pdf或密度。
注1:混合分布的定义是cdf至少表现出一个不连续(或跳跃),并且在至少一个值区间内严格递增。
注2:优先权可以写成“$\theta \sim \ldots$,”这样的语句,其中“$\sim$”表示“分布为”,“…”表示相关分布。同样,数据的模型也可以通过编写“$(y \mid \theta) \sim \ldots$ ..”这样的语句来指定。
注3:在这个阶段,我们通常不会区分$y$作为随机变量和$y$作为该随机变量的值;但有时我们可以使用$Y$表示前者。$y$和$\theta$中的每一个都可以是标量、向量、矩阵或数组。此外,$y$和$\theta$的每个分量可能具有离散分布、连续分布或混合分布。
在下面的前几个示例中,我们将关注最简单的情况,其中$y$和$\theta$都是标量且离散的。
统计代写|贝叶斯分析代考Bayesian Analysis代写|The posterior distribution
贝叶斯推理需要确定$\theta$的后验概率分布。这个任务相当于找到$\theta$的后验pdf,可以使用这个方程来完成
$$
f(\theta \mid y)=\frac{f(\theta) f(y \mid \theta)}{f(y)}
$$
这里,$f(y)$是$y$的无条件(或先前)pdf,由
$$
f(y)=\int f(y \mid \theta) d F(\theta)= \begin{cases}\int f(\theta) f(y \mid \theta) d \theta & \text { if } \theta \text { is continuous } \ \sum_\theta f(\theta) f(y \mid \theta) & \text { if } \theta \text { is discrete. }\end{cases}
$$
注意:在这里,$\int f(y \mid \theta) d F(\theta)$是一个Lebesgue-Stieltjes积分,在$\theta$具有混合分布的情况下,可能需要通过将积分分成两部分来评估。在连续的情况下,把$d F(\theta)$看作 $\frac{d F(\theta)}{d \theta} d \theta=f(\theta) d \theta$

统计代写|贝叶斯分析代考Bayesian Analysis代写 请认准exambang™. exambang™为您的留学生涯保驾护航。
微观经济学代写
微观经济学是主流经济学的一个分支,研究个人和企业在做出有关稀缺资源分配的决策时的行为以及这些个人和企业之间的相互作用。my-assignmentexpert™ 为您的留学生涯保驾护航 在数学Mathematics作业代写方面已经树立了自己的口碑, 保证靠谱, 高质且原创的数学Mathematics代写服务。我们的专家在图论代写Graph Theory代写方面经验极为丰富,各种图论代写Graph Theory相关的作业也就用不着 说。
线性代数代写
线性代数是数学的一个分支,涉及线性方程,如:线性图,如:以及它们在向量空间和通过矩阵的表示。线性代数是几乎所有数学领域的核心。
博弈论代写
现代博弈论始于约翰-冯-诺伊曼(John von Neumann)提出的两人零和博弈中的混合策略均衡的观点及其证明。冯-诺依曼的原始证明使用了关于连续映射到紧凑凸集的布劳威尔定点定理,这成为博弈论和数学经济学的标准方法。在他的论文之后,1944年,他与奥斯卡-莫根斯特恩(Oskar Morgenstern)共同撰写了《游戏和经济行为理论》一书,该书考虑了几个参与者的合作游戏。这本书的第二版提供了预期效用的公理理论,使数理统计学家和经济学家能够处理不确定性下的决策。
微积分代写
微积分,最初被称为无穷小微积分或 “无穷小的微积分”,是对连续变化的数学研究,就像几何学是对形状的研究,而代数是对算术运算的概括研究一样。
它有两个主要分支,微分和积分;微分涉及瞬时变化率和曲线的斜率,而积分涉及数量的累积,以及曲线下或曲线之间的面积。这两个分支通过微积分的基本定理相互联系,它们利用了无限序列和无限级数收敛到一个明确定义的极限的基本概念 。
计量经济学代写
什么是计量经济学?
计量经济学是统计学和数学模型的定量应用,使用数据来发展理论或测试经济学中的现有假设,并根据历史数据预测未来趋势。它对现实世界的数据进行统计试验,然后将结果与被测试的理论进行比较和对比。
根据你是对测试现有理论感兴趣,还是对利用现有数据在这些观察的基础上提出新的假设感兴趣,计量经济学可以细分为两大类:理论和应用。那些经常从事这种实践的人通常被称为计量经济学家。
Matlab代写
MATLAB 是一种用于技术计算的高性能语言。它将计算、可视化和编程集成在一个易于使用的环境中,其中问题和解决方案以熟悉的数学符号表示。典型用途包括:数学和计算算法开发建模、仿真和原型制作数据分析、探索和可视化科学和工程图形应用程序开发,包括图形用户界面构建MATLAB 是一个交互式系统,其基本数据元素是一个不需要维度的数组。这使您可以解决许多技术计算问题,尤其是那些具有矩阵和向量公式的问题,而只需用 C 或 Fortran 等标量非交互式语言编写程序所需的时间的一小部分。MATLAB 名称代表矩阵实验室。MATLAB 最初的编写目的是提供对由 LINPACK 和 EISPACK 项目开发的矩阵软件的轻松访问,这两个项目共同代表了矩阵计算软件的最新技术。MATLAB 经过多年的发展,得到了许多用户的投入。在大学环境中,它是数学、工程和科学入门和高级课程的标准教学工具。在工业领域,MATLAB 是高效研究、开发和分析的首选工具。MATLAB 具有一系列称为工具箱的特定于应用程序的解决方案。对于大多数 MATLAB 用户来说非常重要,工具箱允许您学习和应用专业技术。工具箱是 MATLAB 函数(M 文件)的综合集合,可扩展 MATLAB 环境以解决特定类别的问题。可用工具箱的领域包括信号处理、控制系统、神经网络、模糊逻辑、小波、仿真等。