如果你也在 怎样代写时间序列分析代写Time Series Analysis这个学科遇到相关的难题,请随时右上角联系我们的24/7代写客服。时间序列分析代写Time Series Analysis在数学中,是按时间顺序索引(或列出或绘制)的一系列数据点。最常见的是,一个时间序列是在连续的等距的时间点上的一个序列。因此,它是一个离散时间数据的序列。时间序列的例子是海洋潮汐的高度、太阳黑子的数量和道琼斯工业平均指数的每日收盘值。
时间序列分析代写Time Series Analysis包括分析时间序列数据的方法,以提取有意义的统计数据和数据的其他特征。时间序列预测是使用一个模型来预测基于先前观察到的值的未来值。虽然经常采用回归分析的方式来测试一个或多个不同时间序列之间的关系,但这种类型的分析通常不被称为 “时间序列分析”,它特别指的是单一序列中不同时间点之间的关系。中断的时间序列分析是用来检测一个时间序列从之前到之后的演变变化,这种变化可能会影响基础变量。
my-assignmentexpert™ 时间序列分析代写Time Series Analysis作业代写,免费提交作业要求, 满意后付款,成绩80\%以下全额退款,安全省心无顾虑。专业硕 博写手团队,所有订单可靠准时,保证 100% 原创。my-assignmentexpert™, 最高质量的时间序列分析代写Time Series Analysis作业代写,服务覆盖北美、欧洲、澳洲等 国家。 在代写价格方面,考虑到同学们的经济条件,在保障代写质量的前提下,我们为客户提供最合理的价格。 由于统计Statistics作业种类很多,同时其中的大部分作业在字数上都没有具体要求,因此时间序列分析代写Time Series Analysis作业代写的价格不固定。通常在经济学专家查看完作业要求之后会给出报价。作业难度和截止日期对价格也有很大的影响。
想知道您作业确定的价格吗? 免费下单以相关学科的专家能了解具体的要求之后在1-3个小时就提出价格。专家的 报价比上列的价格能便宜好几倍。
my-assignmentexpert™ 为您的留学生涯保驾护航 在数学Mathematics作业代写方面已经树立了自己的口碑, 保证靠谱, 高质且原创的数学Mathematics代写服务。我们的专家在时间序列分析代写Time Series Analysis代写方面经验极为丰富,各种时间序列分析代写Time Series Analysis相关的作业也就用不着 说。
我们提供的时间序列分析代写Time Series Analysis及其相关学科的代写,服务范围广, 其中包括但不限于:
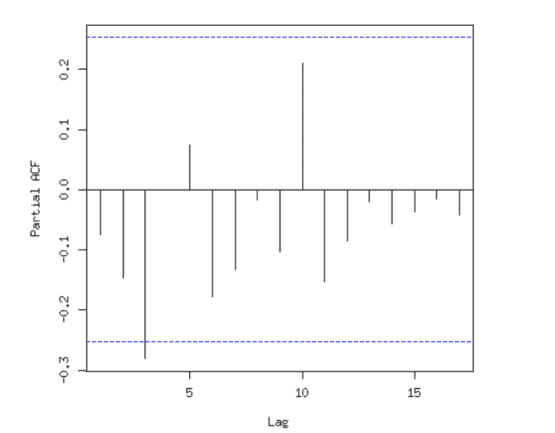
数学代写|时间序列分析代写Time Series Analysis代考|State Space Models: Pluses and Minuses
State space models can be used for both deterministic and stochastic applications, and they can be applied to both continuously sampled data and discretely sampled data. $^{1}$
This alone gives you some inkling of their utility and tremendous flexibility. The flexibility of state space models is what drives both the advantages and disadvantages of this class of models.
There are many strengths of a state space model. A state space model allows for modeling what is often most interesting in a time series: the dynamic process and states producing the noisy data being analyzed, rather than just the noisy data itself. With a state space model, we inject a model of causality into the modeling process to explain what is generating a process in the first place. This is useful for cases where we have strong theories or reliable knowledge about how a system works, and where we want our model to help us suss out more details about general dynamics with which we are already familiar.
A state space model allows for changing coefficients and parameters over time, which means that it allows for changing behavior over time. We did not impose a condition of stationarity on our data when using state space models. This is quite different from the models we examined in Chapter 6, in which a stable process is assumed and modeled with only one set of coefficients rather than time-varying coefficients.
Nonetheless, there are also some disadvantages to a state space model, and sometimes the strength of the state space model is also its weakness:
- Because state space models are so flexible, there are many parameters to be set and many forms a state space model can take. This means that the properties of a particular state space model have often not been well studied. When you formulate a state space model tailored to your time series data, you will rarely have statistical textbooks or academic research papers in which others have studied the model too. This leaves you in less certain territory as far as understanding how your model performs or where you may have committed errors.
- State space models can be very taxing computationally because there are many parameters. Also, the very high number of parameters for some kinds of state space models can leave you vulnerable to overfitting, particularly if you don’t have much data.
数学代写|时间序列分析代写Time Series Analysis代考|The Kalman Filter
The Kalman filter is a well-developed and widely deployed method for incorporating new information from a time series and incorporating it in a smart way with previously known information to estimate an underlying state. One of first uses of the Kalman filter was on the Apollo 11 mission-the filter was chosen when NASA engineers realized that the onboard computing elements would not allow other, more memory-intensive techniques of position estimation. As you will see in this section, the benefits of the Kalman filter are that it is relatively easy to compute and does not require storage of past data to make present estimates or future forecasts.
Overview
The mathematics of the Kalman filter can be intimidating to a newcomer, not because it is especially difficult but because there are a fair number of quantities to keep track of, and it’s an iterative, somewhat circular process with many related quantities. For this reason, we will not derive the Kalman filter equations here, but instead go through a high-level overview of those equations to get a sense of how they work. $^{2}$
We begin with a linear Gaussian model, positing that our state and our observations have the following dynamics:
$$
\begin{aligned}
&x_{t}=F \times x_{t-1}+B \times u_{t}+w_{t} \
&y_{t}=A \times x_{t}+v_{t}
\end{aligned}
$$
That is, the state at time $\mathrm{t}$ is a function of the state at the previous time step $\left(F \times x_{t-1}\right)$, an external force term $\left(B \times u_{t}\right)$, and a stochastic term $\left(w_{t}\right)$. Likewise, the measurement at time $t$ is a function of the state at time $t$ and a stochastic error term, measurement error.
数学代写|时间序列分析代写TIME SERIES ANALYSIS代考|Hidden Markov Models
Hidden Markov Models (HMMs) are a particularly useful and interesting way of modeling a time series because it is a rare instance of unsupervised learning in time series analysis, meaning there is no labeled correct answer against which to train. An HMM is motivated by an intuition similar to what we used when experimenting with the Kalman filter earlier in this chapter, namely the idea that the variables we are able to observe may not be the most descriptive variables of the system. As with the Kalman filter applied to a linear Gaussian model, we posit the idea that the process has a state, and our observations give information about this state. And again, as before, we need to have some opinion as to how the state variables influence what we can observe. In the case of an HMM, what we posit is that the process is a nonlinear one characterized by jumps between discrete states.
How the Model Works
An HMM posits a system in which there are states that are not directly observable. The system is a Markov process, which means that it is “memoryless” in the sense that the probabilities of future events can be fully calculated given only the system’s current state. That is, knowing the system’s current state and its previous states is no more useful than simply knowing the system’s current state.
Markov processes are often described in terms of matrices. For example, suppose there was a system that fluctuated between state $A$ and state $B$. When in either state, the system was statistically more likely to remain in the same state than to flip to the other state at any distinct time step. One such system would be described by the following matrix:
A B
A 0.7 0.3
B 0.2 0.8
Let’s imagine that our system is in state $A$, namely $(1,0)$. (State B would be $(0,1)$.) In such a case, the probability that the system would remain in state A is $0.7$, whereas the probability that the system would flip is $0.3$. We don’t need to know what states the system was in before its most recent moment in time. This is what it means to be a Markov process.
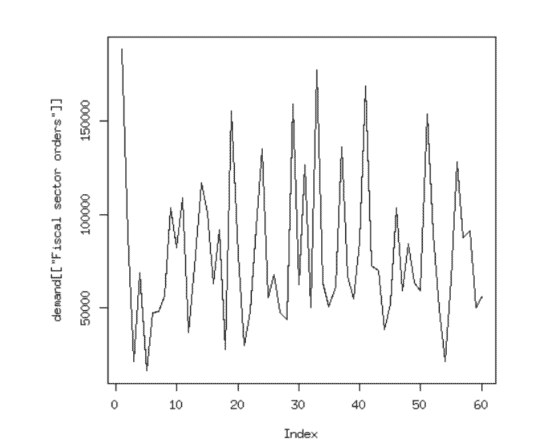
时间序列分析代写
数学代写|时间序列分析代写TIME SERIES ANALYSIS代考|STATE SPACE MODELS: PLUSES AND MINUSES
状态空间模型可用于确定性和随机应用,它们既可以应用于连续采样数据,也可以应用于离散采样数据。1
仅此一项就可以让您对它们的实用性和巨大的灵活性有所了解。状态空间模型的灵活性是驱动此类模型的优点和缺点的原因。
状态空间模型有很多优点。状态空间模型允许对时间序列中通常最有趣的东西进行建模:动态过程和产生被分析的噪声数据的状态,而不仅仅是噪声数据本身。通过状态空间模型,我们将因果关系模型注入到建模过程中,以首先解释是什么产生了过程。这对于我们拥有关于系统如何工作的强大理论或可靠知识以及我们希望我们的模型帮助我们找出我们已经熟悉的一般动力学的更多细节的情况很有用。
状态空间模型允许随时间改变系数和参数,这意味着它允许随时间改变行为。在使用状态空间模型时,我们没有对我们的数据施加平稳性条件。这与我们在第 6 章中研究过的模型完全不同,在第 6 章中,仅使用一组系数而不是随时间变化的系数来假设和建模一个稳定的过程。
尽管如此,状态空间模型也有一些缺点,有时状态空间模型的优势也是它的弱点:
- 由于状态空间模型非常灵活,因此需要设置许多参数,并且状态空间模型可以采用多种形式。这意味着特定状态空间模型的属性通常没有得到很好的研究。当您制定适合您的时间序列数据的状态空间模型时,您将很少有其他人也研究过该模型的统计教科书或学术研究论文。就了解您的模型如何执行或您可能在哪里犯了错误而言,这使您处于不太确定的领域。
- 状态空间模型在计算上可能非常繁重,因为有很多参数。此外,某些状态空间模型的大量参数可能会使您容易受到过度拟合的影响,尤其是在您没有太多数据的情况下。
数学代写|时间序列分析代写TIME SERIES ANALYSIS代考|THE KALMAN FILTER
卡尔曼滤波器是一种成熟且广泛部署的方法,用于合并来自时间序列的新信息,并以智能方式将其与先前已知的信息合并以估计潜在状态。卡尔曼滤波器的第一个用途是在阿波罗 11 号任务中——当 NASA 工程师意识到机载计算元件不允许使用其他更占用内存的位置估计技术时,选择了该滤波器。正如您将在本节中看到的,卡尔曼滤波器的好处是计算相对容易,并且不需要存储过去的数据来进行当前估计或未来预测。
概述
卡尔曼滤波器的数学运算可能会让新手望而却步,不是因为它特别困难,而是因为有相当数量的量需要跟踪,而且它是一个迭代的、有点循环的过程,有许多相关的量。出于这个原因,我们不会在这里推导卡尔曼滤波器方程,而是通过对这些方程的高级概述来了解它们是如何工作的。2
我们从线性高斯模型开始,假设我们的状态和观察具有以下动态:
X吨=F×X吨−1+乙×在吨+在吨 是吨=一种×X吨+在吨
也就是当时的状态吨是上一个时间步的状态的函数(F×X吨−1), 外力项(乙×在吨), 和一个随机项(在吨). 同样,时间测量吨是当时状态的函数吨和一个随机误差项,测量误差。
数学代写|时间序列分析代写TIME SERIES ANALYSIS代考|HIDDEN MARKOV MODELS
隐马尔可夫模型H米米s是一种特别有用和有趣的时间序列建模方法,因为它是时间序列分析中罕见的无监督学习实例,这意味着没有标记的正确答案可供训练。HMM 的动机类似于我们在本章前面试验卡尔曼滤波器时使用的直觉,即我们能够观察到的变量可能不是系统中最具描述性的变量。与应用于线性高斯模型的卡尔曼滤波器一样,我们假设过程具有状态,并且我们的观察提供了有关该状态的信息。同样,和以前一样,我们需要对状态变量如何影响我们可以观察到的内容有一些看法。在 HMM 的情况下,
模型是如何工作
的 HMM 假设了一个系统,其中存在无法直接观察到的状态。该系统是一个马尔可夫过程,这意味着它是“无记忆的”,即仅给定系统的当前状态就可以完全计算未来事件的概率。也就是说,知道系统的当前状态和它以前的状态并不比简单地知道系统的当前状态有用。
马尔可夫过程通常用矩阵来描述。例如,假设有一个系统在状态之间波动一种和状态乙. 在任一状态下,系统在统计上更有可能保持在同一状态,而不是在任何不同的时间步切换到另一个状态。一个这样的系统将由以下矩阵描述:
AB
A 0.7 0.3
B 0.2 0.8
假设我们的系统处于状态一种,即(1,0). 小号吨一种吨和乙在这在ldb和$(0,1.)一世ns在CH一种C一种s和,吨H和pr这b一种b一世l一世吨是吨H一种吨吨H和s是s吨和米在这在ldr和米一种一世n一世ns吨一种吨和一种一世s0.7,在H和r和一种s吨H和pr这b一种b一世l一世吨是吨H一种吨吨H和s是s吨和米在这在ldFl一世p一世s0.3 美元。我们不需要知道系统在其最近时刻之前处于什么状态。这就是马尔可夫过程的含义。
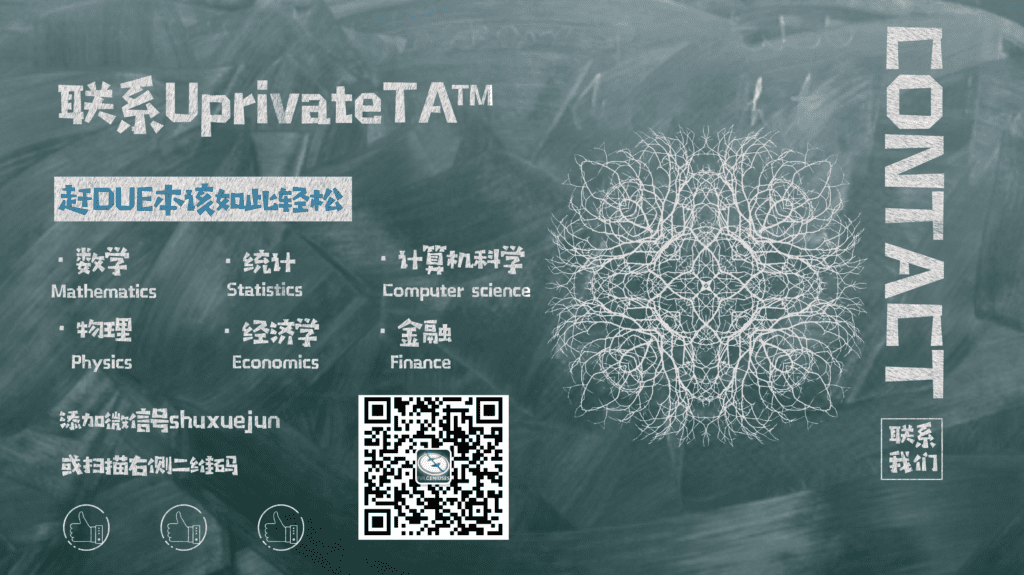
数学代写|时间序列分析代写Time Series Analysis代考 请认准UprivateTA™. UprivateTA™为您的留学生涯保驾护航。
电磁学代考
物理代考服务:
物理Physics考试代考、留学生物理online exam代考、电磁学代考、热力学代考、相对论代考、电动力学代考、电磁学代考、分析力学代考、澳洲物理代考、北美物理考试代考、美国留学生物理final exam代考、加拿大物理midterm代考、澳洲物理online exam代考、英国物理online quiz代考等。
光学代考
光学(Optics),是物理学的分支,主要是研究光的现象、性质与应用,包括光与物质之间的相互作用、光学仪器的制作。光学通常研究红外线、紫外线及可见光的物理行为。因为光是电磁波,其它形式的电磁辐射,例如X射线、微波、电磁辐射及无线电波等等也具有类似光的特性。
大多数常见的光学现象都可以用经典电动力学理论来说明。但是,通常这全套理论很难实际应用,必需先假定简单模型。几何光学的模型最为容易使用。
相对论代考
上至高压线,下至发电机,只要用到电的地方就有相对论效应存在!相对论是关于时空和引力的理论,主要由爱因斯坦创立,相对论的提出给物理学带来了革命性的变化,被誉为现代物理性最伟大的基础理论。
流体力学代考
流体力学是力学的一个分支。 主要研究在各种力的作用下流体本身的状态,以及流体和固体壁面、流体和流体之间、流体与其他运动形态之间的相互作用的力学分支。
随机过程代写
随机过程,是依赖于参数的一组随机变量的全体,参数通常是时间。 随机变量是随机现象的数量表现,其取值随着偶然因素的影响而改变。 例如,某商店在从时间t0到时间tK这段时间内接待顾客的人数,就是依赖于时间t的一组随机变量,即随机过程
Matlab代写
MATLAB 是一种用于技术计算的高性能语言。它将计算、可视化和编程集成在一个易于使用的环境中,其中问题和解决方案以熟悉的数学符号表示。典型用途包括:数学和计算算法开发建模、仿真和原型制作数据分析、探索和可视化科学和工程图形应用程序开发,包括图形用户界面构建MATLAB 是一个交互式系统,其基本数据元素是一个不需要维度的数组。这使您可以解决许多技术计算问题,尤其是那些具有矩阵和向量公式的问题,而只需用 C 或 Fortran 等标量非交互式语言编写程序所需的时间的一小部分。MATLAB 名称代表矩阵实验室。MATLAB 最初的编写目的是提供对由 LINPACK 和 EISPACK 项目开发的矩阵软件的轻松访问,这两个项目共同代表了矩阵计算软件的最新技术。MATLAB 经过多年的发展,得到了许多用户的投入。在大学环境中,它是数学、工程和科学入门和高级课程的标准教学工具。在工业领域,MATLAB 是高效研究、开发和分析的首选工具。MATLAB 具有一系列称为工具箱的特定于应用程序的解决方案。对于大多数 MATLAB 用户来说非常重要,工具箱允许您学习和应用专业技术。工具箱是 MATLAB 函数(M 文件)的综合集合,可扩展 MATLAB 环境以解决特定类别的问题。可用工具箱的领域包括信号处理、控制系统、神经网络、模糊逻辑、小波、仿真等。